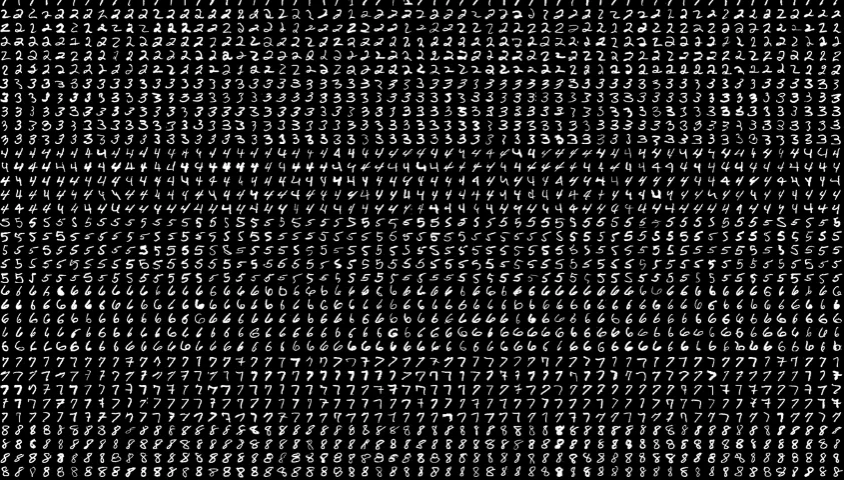
Was ist ein Convolutional Neural Network?
Dr. Mark Bugden
dida entwickelt kundenspezifische Computer-Vision-Lösungen, beteiligt sich aktiv an KI-Forschung und veröffentlicht regelmäßig technische Inhalte zu unterschiedlichen Aspekten im Bereich Computer Vision.
Dr. Mark Bugden
Tiago Sanona
Edit Szügyi
Emilius Richter
Madina Kasymova
Felix Brunner
Dr. Frank Weilandt
Konrad Mundinger
Fabian Gringel
Moritz Besser
Dmitrii Iakushechkin
Fabian Dechent
Dr. Augusto Stoffel
Tiago Sanona
Johan Dettmar
Matthias Werner
Dr. William Clemens
Dr. William Clemens
Fabian Gringel
Dr. William Clemens
Lorenzo Melchior
Matthias Werner
Dr. Nelson Martins
Wie Machine Learning dazu beitragen kann, Umweltzerstörung zu verhindern.
Für den DWD haben wir die Erkennung bestimmter Wolkenarten automatisiert.
Ein Algorithmus zur Bildsegmentierung unterstützt nachhaltige Stadtplanung.
Wir erkennen angebaute Feldfrüchte anhand von Satellitendaten, um die Landwirtschaft zu unterstützen.
Machine Learning in der Produktion: Entwicklung einer KI-gestützten Software zur optischen Defekterkennung für die Halbleiterlaserproduktion
Für eine automatische Zugangskontrolle entwickelten wir ein mehrstufiges Sicherheitssystem mit Gesichtserkennung.
AI-basierte Erkennung von Düsenverschmutzung im industriellen 3D-Druck
Automatische Erkennung gefährlicher Objekte in Videoaufnahmen von Zügen.
Unter Verwendung verschiedener Satellitenbilder wird eine Reihe von Computer-Vision-Modellen und mathematischer Modellierung angewandt, um Abraumhalden zu erkennen, zu segmentieren und hinsichtlich ihres Volumens und ihres mineralogischen Gehalts zu analysieren.
Durch kreative Lösungen konnten wir die Planung von Solaranlagen automatisieren.
Frank Weilandt, Robert Behling, Romulo Goncalves, Arash Madadi, Lorenz Richter, Tiago Sanona, Daniel Spengler, Jona Welsch
In this article, we propose a deep learning-based algorithm for the classification of crop types from Sentinel-1 and Sentinel-2 time series data which is based on the celebrated transformer architecture. Crucially, we enable our algorithm to do early classification, i.e., predict crop types at arbitrary time points early in the year with a single trained model (progressive intra-season classification). Such early season predictions are of practical relevance for instance for yield forecasts or the modeling of agricultural water balances, therefore being important for the public as well as the private sector. Furthermore, we improve the mechanism of combining different data sources for the prediction task, allowing for both optical and radar data as inputs (multi-modal data fusion) without the need for temporal interpolation. We can demonstrate the effectiveness of our approach on an extensive data set from three federal states of Germany reaching an average F1 score of 0.920.92 using data of a complete growing season to predict the eight most important crop types and an F1 score above 0.80.8 when doing early classification at least one month before harvest time. In carefully chosen experiments, we can show that our model generalizes well in time and space.
Theophil Trippe, Martin Genzel, Jan Macdonald, Maximilian März
This work presents a novel deep-learning-based pipeline for the inverse problem of image deblurring, leveraging augmentation and pre-training with synthetic data. Our results build on our winning submission to the recent Helsinki Deblur Challenge 2021, whose goal was to explore the limits of state-of-the-art deblurring algorithms in a real-world data setting.
Martin Genzel, Jan Macdonald, Maximilian März
In the past five years, deep learning methods have become state-of-the-art in solving various inverse problems. Before such approaches can find application in safety-critical fields, a verification of their reliability appears mandatory. Recent works have pointed out instabilities of deep neural networks for several image reconstruction tasks.
Christof Zink, Michael Ekterai, Dominik Martin, William Clemens, Angela Maennel, Konrad Mundinger, Lorenz Richter, Paul Crump, Andrea Knigge
The optical inspection of the surfaces of diode lasers, especially the p-sides and facets, is an essential part of the quality control in the laser fabrication procedure. With reliable, fast, and flexible optical inspection processes, it is possible to identify and eliminate defects, accelerate device selection, reduce production costs, and shorten the cycle time for product development.
Martin Genzel, Ingo Gühring, Jan Macdonald, Maximilian März
This work is concerned with the following fundamental question in scientific machine learning: Can deep-learning-based methods solve noise-free inverse problems to near-perfect accuracy? Positive evidence is provided for the first time, focusing on a prototypical computed tomography (CT) setup.
Luis Oala, Cosmas Heiß, Jan Macdonald, Maximilian März, Gitta Kutyniok, Wojciech Samek
The quantitative detection of failure modes is important for making deep neural networks reliable and usable at scale. We consider three examples for common failure modes in image reconstruction and demonstrate the potential of uncertainty quantification as a fine-grained alarm system.
Jan Macdonald, Maximilian März, Luis Oala, Wojciech Samek
This work investigates the detection of instabilities that may occur when utilizing deep learning models for image reconstruction tasks. Although neural networks often empirically outperform traditional reconstruction methods, their usage for sensitive medical applications remains controversial.
Jan Macdonald, Lars Ruthotto
We present an improved technique for susceptibility artifact correction in echo-planar imaging (EPI), a widely used ultra-fast magnetic resonance imaging (MRI) technique. Our method corrects geometric deformations and intensity modulations present in EPI images.
Björn Sbierski, Maximilian Trescher, Emil J. Bergholtz, Piet W. Brouwer
Double Weyl nodes are topologically protected band crossing points which carry chiral charge ±2. They are stabilized by C4 point-group symmetry and are predicted to occur in SrSi2 or HgCr2Se4. We study their stability and physical properties in the presence of a disorder potential.
dida webinar
Moritz Besser & Jona Welsch
Moritz Besser und Jona Welsch
6. Dezember 2021
dida webinar
William Clemens
William Clemens
26. Februar 2020