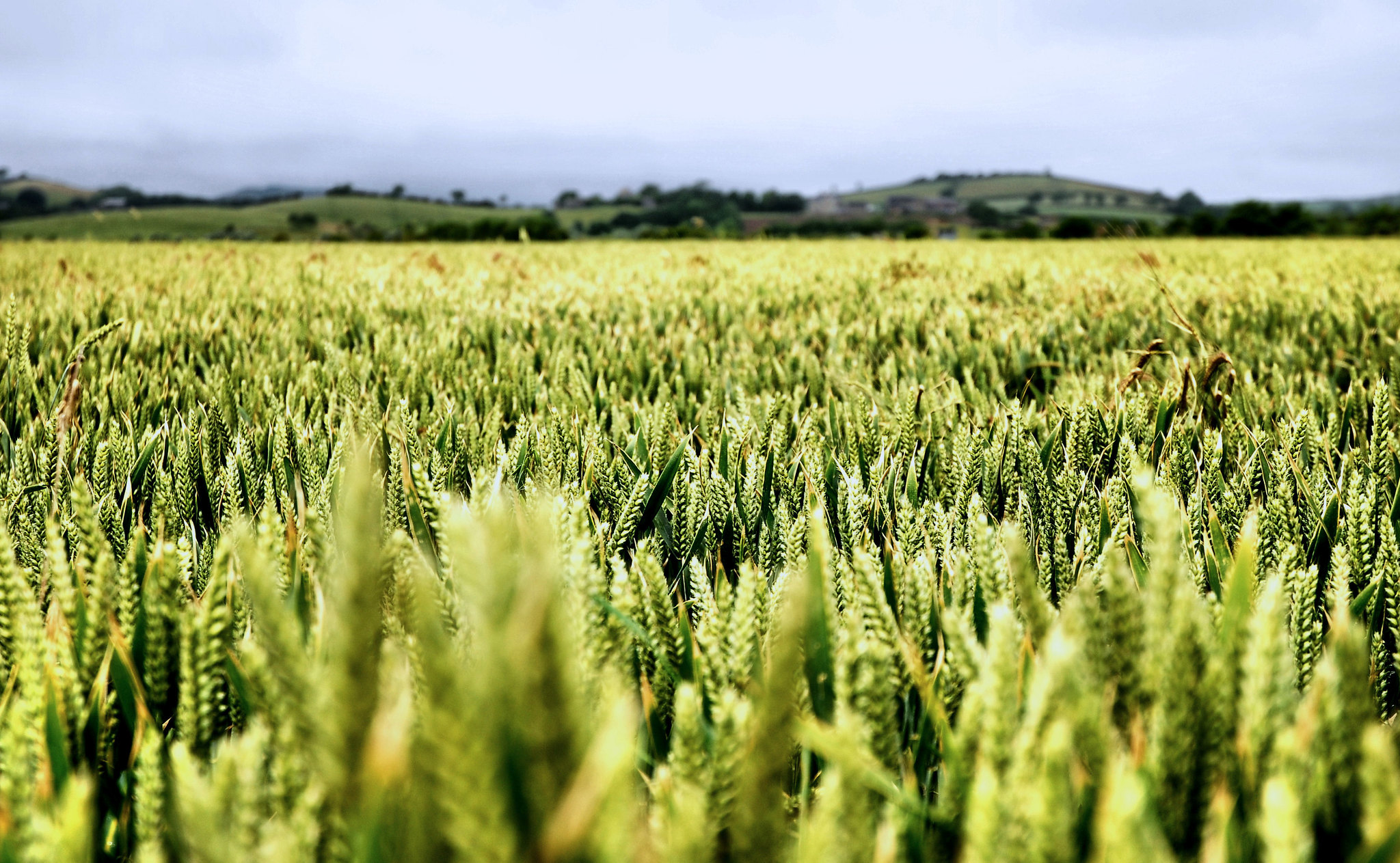
Early Classification of Crop Fields through Satellite Image Time Series
Tiago Sanona
In a fast paced and always changing global economy the ability to classify crop fields via remote sensing at the end of a growth cycle does not provide the much needed immediate insight required by decision makers. To address this problem we developed a model that allows continuous classification of crop fields at any point in time and improves predictions as more data becomes available. In practice, we developed a single model capable of delivering predictions about which crops are growing at any point in time based on satellite data. The data available at the time of inference could be a few images at the beginning of the year or a full time series of images from a complete growing cycle. This exceeds the capabilities of current deep learning solutions that either only offer predictions at the end of the growing cycle or have to use multiple models that are specialized to return results from pre-specified points in time. This article details the key changes we employed to the model described in a previous blog post “Classification of Crop fields through Satellite Image Time Series” that enlarges its functionality and performance. The results presented in this article are based on a research paper recently published by dida. For more detailed information about this topic and other experiments on this model please check out the original manuscript: “Early Crop Classification via Multi-Modal Satellite Data Fusion and Temporal Attention” .