AI Index Report 2022: key findings about the status quo of AI
David Berscheid
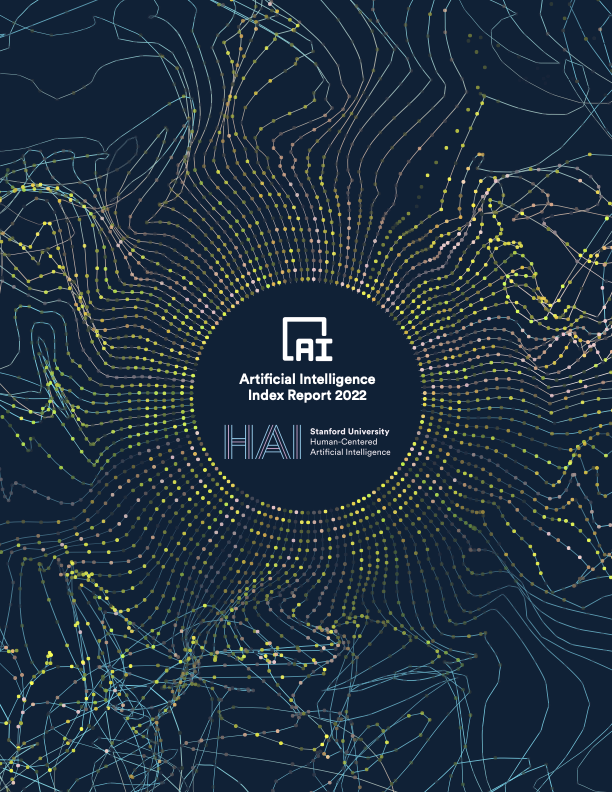
The AI Index Report tracks and collects data regarding the worldwide development of artificial intelligence (AI). This years fifth edition, by the independent initiative at the Stanford Institute for Human-Centered Artificial Intelligence (HAI), is again aimed at informing relevant stakeholders like policy makers, researcher or related industries about the enormous advances of AI, the technological and societal stages of most prominent AI disciplines, as well as creating awareness for arising problems.
In this article, we will discuss a selection of the report’s machine learning (ML)-related key messages as well as respectively add dida’s perspective to the following topics:
Research and Development
Technical Performance
Technical AI Ethics
The Economy and Education
AI Policy and Governance
For the full report please visit the original source here.
Research and Development
Research and Development (R&D) players in the field of ML are as active and connected (by countries and sectors ) as never before.
In 2022, many daily services inhibit ML-related functionalities that originally stem from findings of public ML communities and their R&D activities. These communities work across borders and sectors, and largely open source their results. Thus, the public has access to highly valuable contributions, leading therefore to rapid advances in ML technologies.
Looking at R&D activities by nations, it becomes clear that the two most relevant nations are the United States of America and China. While China is producing the highest number of publications per year, the US achieves the highest number of citations. Overall, the number of publications doubled from 2010 to 2021 (to approx. 334K). Amongst them, are many publications as a result of international collaborations between two countries. Here again, the collaboration between the US and China produces most results, followed by the UK and China. Germany ranks 5th with its collaboration partner the US.
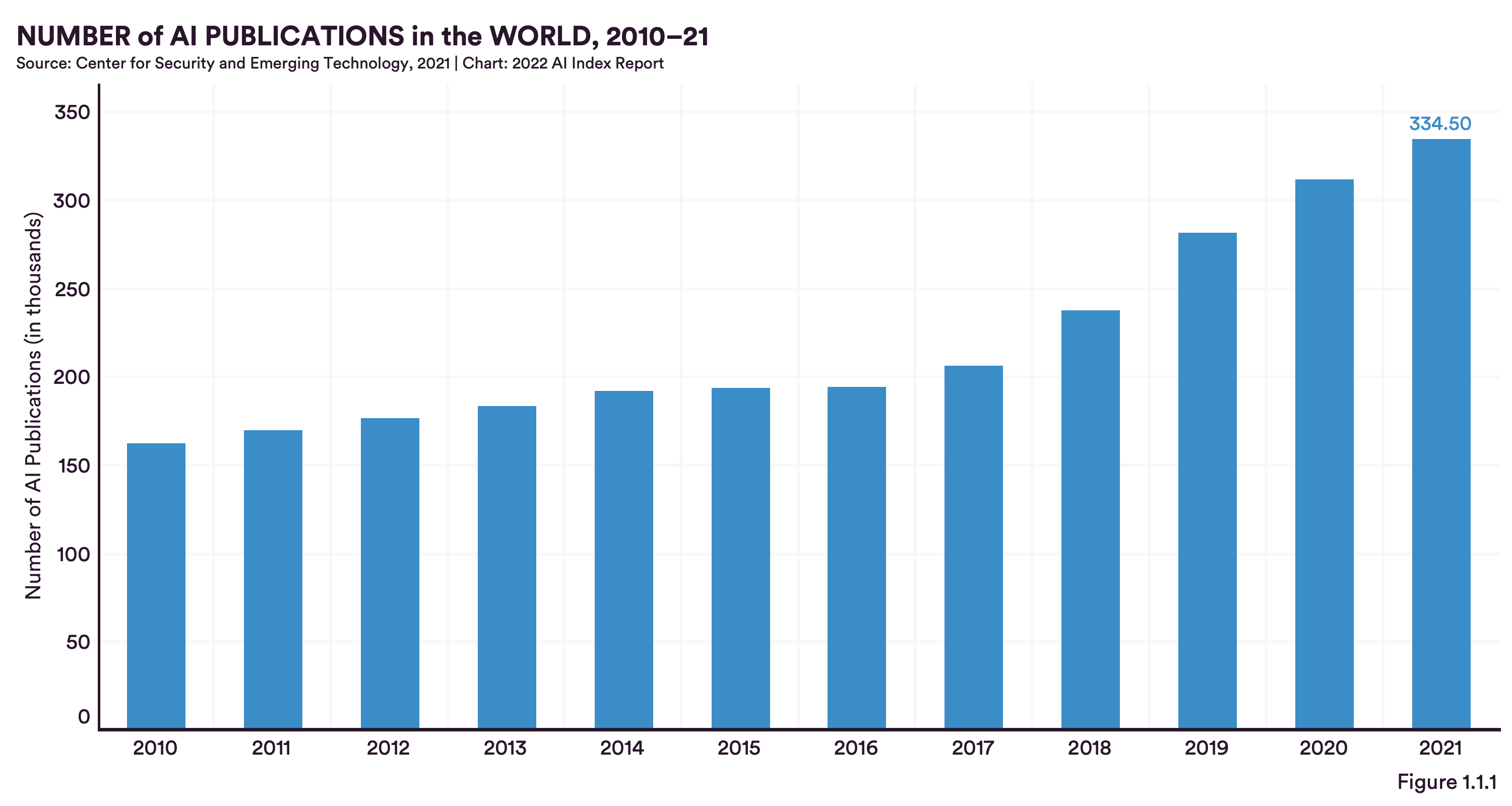
The most relevant mediums for publishing research findings are academic journals, then conferences and then code repositories, whereas book-related publications are less important in AI research. When ranking AI conferences by their attendance, the two most renowned ones remain NeurIPS and ICML. At dida we also try to actively engage in ML research activities, which we then transfer into production software, thus bridging the gap between academia and industry. We are glad that we could already contribute papers to both conferences (NeurIPS in 2020 and an ‘outstanding paper’ to the ICML in 2021), and thereby participate in the global research community.
Technical Performance
Technical performances improved significantly within one year, pushing solutions closer to more general abilities and industry deployments, while at the same time ML’s weak points are growing likewise.
In order to compare technical performances of different ML solutions, depending on the specific task, they are typically tested on a few huge and open source data sets, like ImageNet for image classification tasks or SQuAD for question answering tasks. One trend that keeps succeeding against existing benchmark solutions is the inclusion of external training data in CV and NLP projects (9 out of 10 ML solutions that could beat existing benchmarks make use of this). At dida, we agree and have also already discussed the importance of data for our solutions, explaining how a data-centric approach can improve performances.
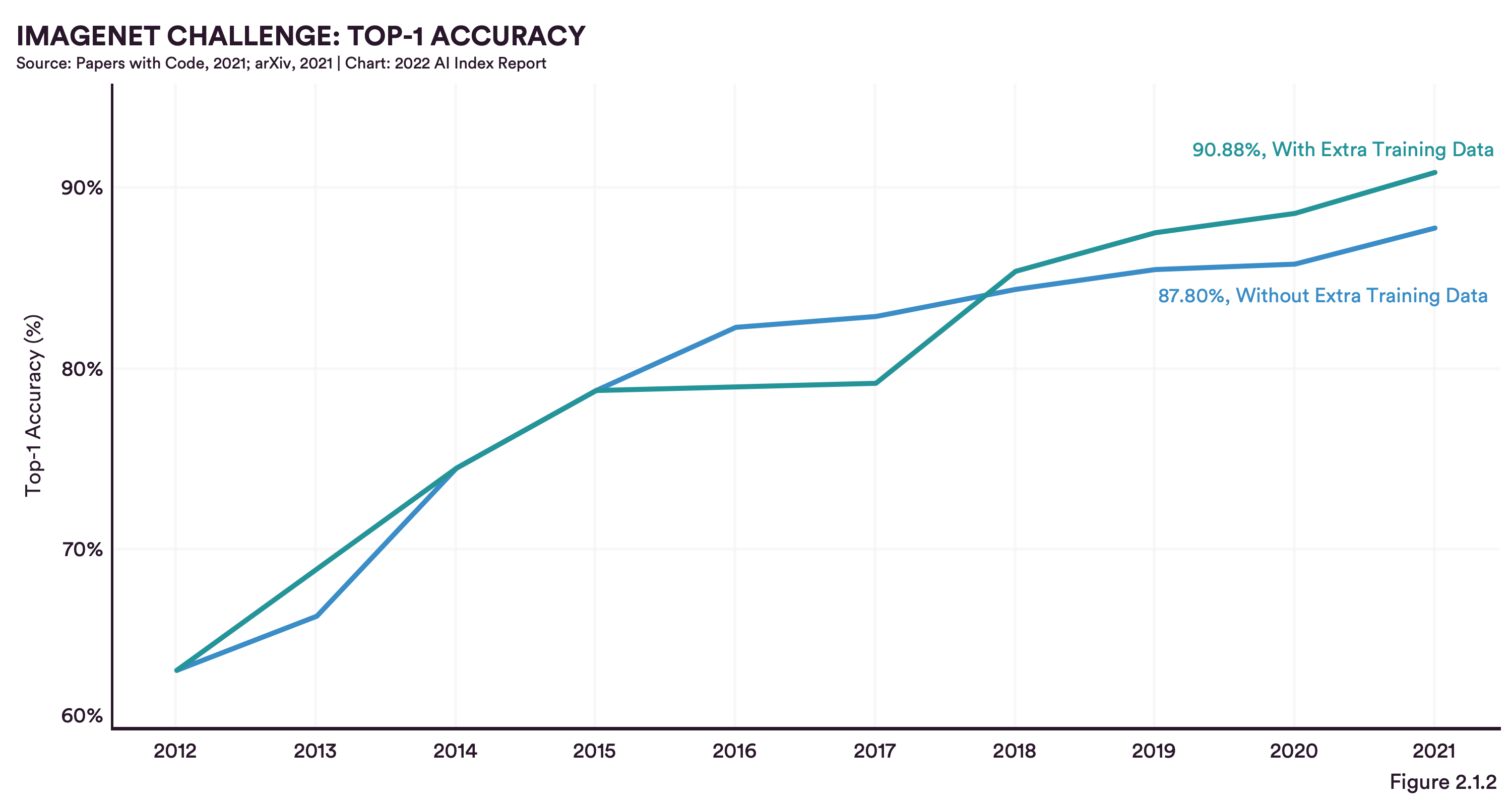
The quintessence of the report’s chapter on computer vision is basically that in all subfields of CV (i.e. image classification, image generation, pose estimation, face detection) performance curves are constantly increasing, even though not as extreme as in previous years, or as for younger subfields of ML, like reinforcement learning. According to the authors, CV solutions today are already highly capable and convolutional neural networks (CNNs) the established architecture of choice, so that improvements are rather focussed on details than on completely new approaches.
Surprisingly, the report does not present numbers regarding the promising development of visual transformer models (ViTs) in the context of CV, which we at dida consider very useful. It will likely receive more attention in the next report.
In general, our perspective at dida aligns with the report's findings that CV reached a level of maturity, where the focus shifted more to deployment and away from foundational research. Adding to that, dida's key contributions in past projects like ASMspotter or Crop Type Classification were less about the general model architectures but more about mathematical details in the pre- and post-processing, data quality or making use of the time dimension. If you are interested in ML solutions for remote sensing data feel free to check out our related webinar.
Natural Language Processing (NLP) contains a set of subtasks like language understanding, text summarization, natural language interference, sentiment analysis or machine translation - some more complex than others. These NLP models are typically compared to the capabilities of humans on the exact same task. While ML already outperforms humans for basic tasks, like reading comprehension, ML models are still weaker for more complex tasks, such as abductive natural language inference (the capability of common sense reasoning based on only pieces of information). Nevertheless, language models are becoming more capable than ever, also largely due to the rise of transformer models, like BERT or GPT-3, which can be seen as the de facto standard in NLP (similarly to what CNNs have been for CV in the last years).
Despite its powerful capabilities, transformer models are quite large and can hardly be trained from scratch, which on the one hand means that for projects with only small data sets, approaches based on recurrent neural networks (RNN), like LSMTs can still be preferable, and on the other hand leads to the following weakness: NLP solutions heavily depend on pre-trained models that make working with text data more efficient and in many cases possible at all. While the open source availability to pre-trained ML models is a great benefit for the whole ML community, it also comes with risks, as research showed also that the largest, most popular and most advanced language models are not free of biases. But because of their benefits, NLP solutions can hardly work without them. The index AI report therefore dedicates a complete chapter to the young research field of AI ethics.
In contrast to the more mature areas of CV and NLP, reinforcement learning (RL) algorithms have gone through a faster development. While in earlier years they improved on very specific tasks, like playing the game of go, this year's AI Index Report states that they are becoming increasingly good at more general skills. The RL environment Procgen by OpenAI demonstrates that very well as its RL capabilities are tested on a set of 16 different Procgen games instead of only one. With this development, it looks like RL is starting to follow the path of CV and NLP, which also evolved from specific to more general solutions, followed by widespread deployments. Future challenges will now be to create solutions that are efficient on top of that.
Technical AI ethics
Increasingly complex biases are evolving through more complex ML models, which will pose a challenge for academia and industry.
In the chapter ‘Technical AI ethics’ the AI Index report 2022 presents data on the development of the field of AI ethics, its cause, importance and outlook for the future. Generally, algorithms’ predictions are considered to be fair, when the predictions do not positively or negatively influence groups based on attributes like gender, ethnicity or religion. But because research could show that there are several types of biases in today's ML models, more and more publications (from academia but also industry) now focus on ethical aspects.
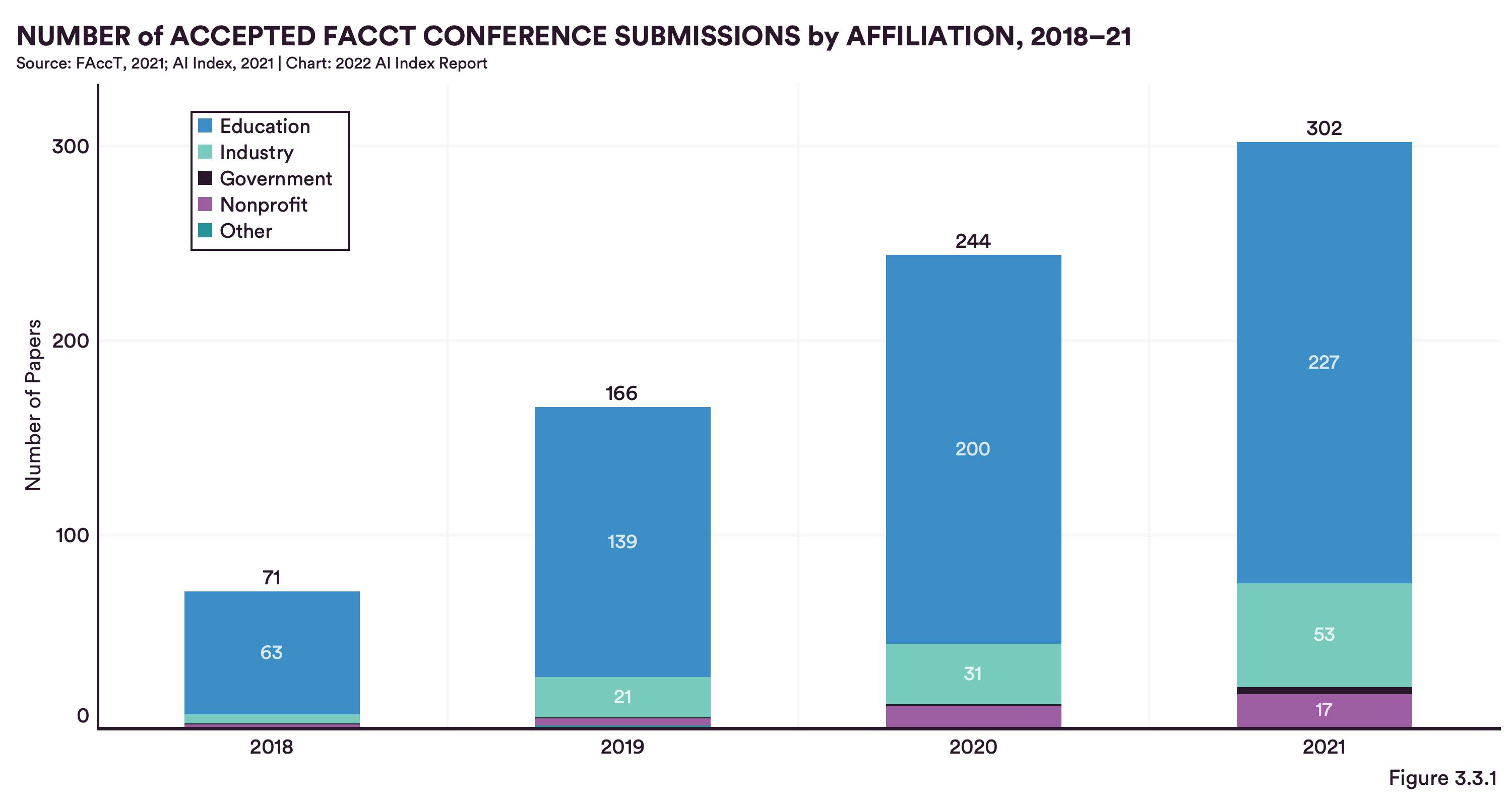
In language models for example, it could be shown that the more complex a model is, the more likely it is to produce toxic outputs, when prompted with toxic inputs. At the same time though, more complex models are better at detecting toxicity in their own outputs. The report states that efforts to reduce toxicity up until now lead to weaker performances of the models. Furthermore, multimodal models (models that make use of multiple input types, like images, texts or speech) seem to produce multimodal biases, and the more complex the models get, the harder it gets to fight these problems. Jack Clarck, Co-director of the AI Index Report, explains that ethical challenges are different to technological ones, as they need a socio-technical approach to solving them and not simply a traditional technological solution, which in his opinion is much harder to find than the solutions ML needed before.
If you are interested in more information regarding ethics and ML, feel free to read our introductory blog article about it.
Economy and Education
Economical and educational signs suggest more and more AI deployments, as more money is being invested in AI than ever before and the amount of AI specialists is rising.
In terms of capital flow, it is interesting to see that the amount of private investments approx. doubled from 2020 to 2021, while the number of newly founded companies decreases, leading to a concentration of capital for less players.
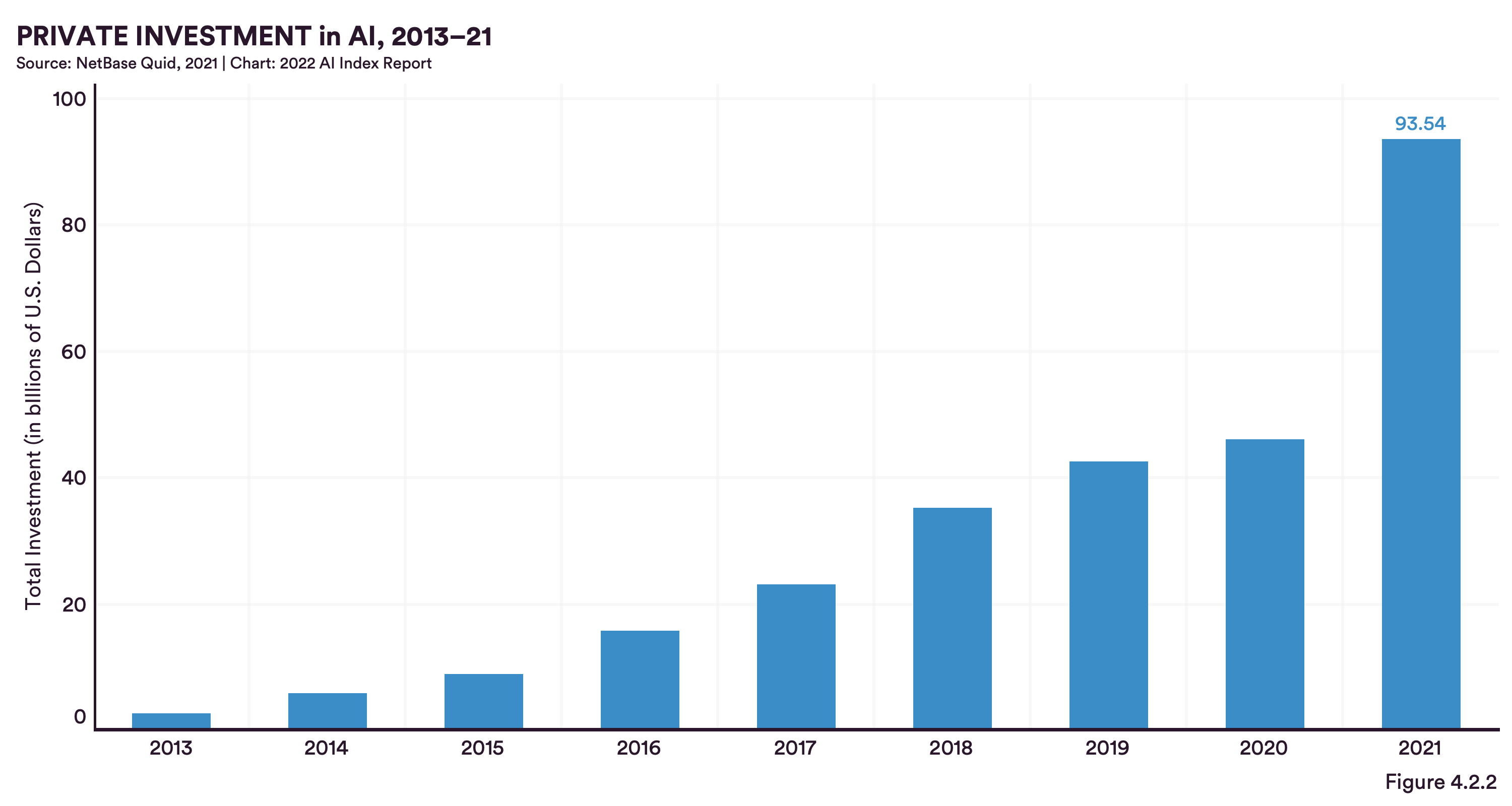
AI is not only attractive for investors but also for job applicants. Data for the US reveals that 1 in every 5 computer science PhDs specializes in AI, making it the most attractive specialization. Most of these graduates then find jobs in the industry as opposed to academia or other organizations. What the AI Index Report does not consider are similar academic fields, where the AI affinity might be equally high or potentially even higher. A quick look at our team at dida reveals that most of our Machine Learning scientists have backgrounds in mathematics or physics - two areas that in our experience produce highly capable AI specialists. Thus, even more AI specialists might be heading towards the AI industry.
AI Policy and Governance
Policy makers and governments are aware of the rapid development of AI and started to discuss potential legislative actions.
Technological developments can typically evolve at a higher pace than political debates and laws. In 2016 legislation slowly started to act and introduce AI-related laws. By 2021 18 laws have globally been passed - Spain, the UK and the US leading the ranking with 3 laws respectively. Even though the passage rate of such laws remains low (i.e. only 3 out of 130 AI bills passed in the US) politics is creating awareness amongst policy makers. Correspondingly the amount of times AI-related content is mentioned during US congressional sessions rises steeply. Thus, the report indicates that legislators are aware of the need for AI-related laws and are increasingly signaling their willingness to act until they will seemingly ultimately do so. More frequent AI laws can therefore be expected, which will stimulate / regulate AI-related industries significantly.
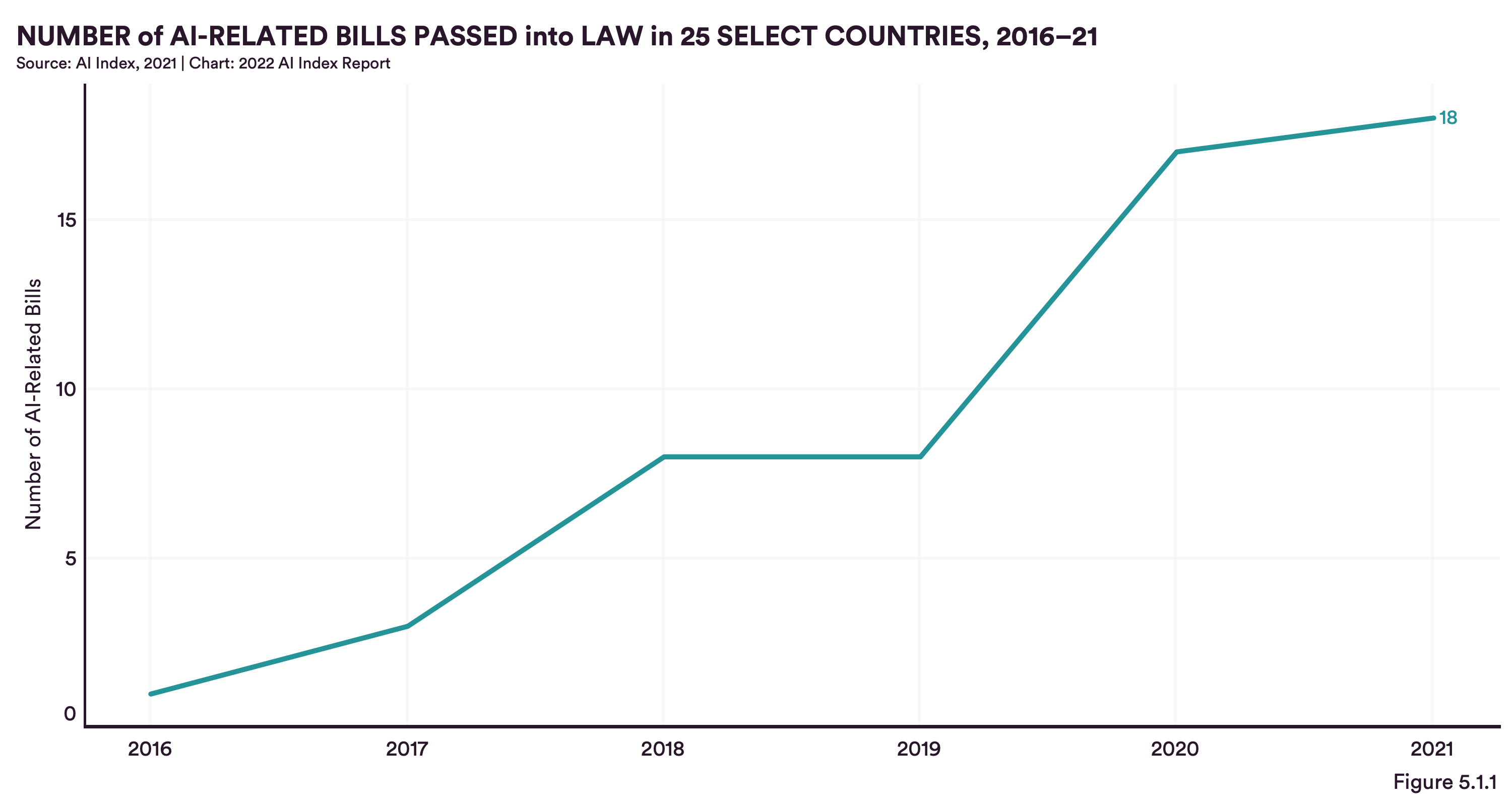
Conclusion
The Index AI report 2022 provides many interesting statistics and insights on the development of AI and related fields. The key messages can be broken down to the following:
R&D efforts are higher than ever and AI seems to have developed from prestige projects to a real priority.
While research was formerly mostly done by academia, industry research is growing and influencing the AI community significantly.
Performances and capabilities of many AI technologies are already at an above-human or close-to-human level that issues of industry deployment and scalability are moving more into the focus than further model improvements.
As AI models are becoming more complex, so are its related ethical problems, which explains the rise of AI ethics research looking to overcome problems of growing biases and discrimination.
More capital than ever is invested into AI solutions while the number of newly founded AI companies is decreasing leading to a capital concentration for existing AI players.
Policy makers around the world are increasingly aware of the need for AI-related laws and while the actual introduction of such laws remains rare, politics is signaling that they will act more and more in the next few years.
Source:
Daniel Zhang, Nestor Maslej, Erik Brynjolfsson, John Etchemendy, Terah Lyons, James Manyika, Helen Ngo, Juan Carlos Niebles, Michael Sellitto, Ellie Sakhaee, Yoav Shoham, Jack Clark, and Raymond Perrault, “The AI Index 2022 Annual Report,” AI Index Steering Committee, Stanford Institute for Human-Centered AI, Stanford University, March 2022.
Contact
If you would like to speak with us about this topic, please reach out and we will schedule an introductory meeting right away.