Context
Thunderstorms and large amounts of precipitation present a major commercial risk to farmers. To prevent harvest damages due to severe weather events, farmers conclude an insurance and pay an insurance premium to be compensated in case of damages. To provide a timely and competent service to farmers, insurance companies need to be able to process claims from severe weather events in a short period of time.
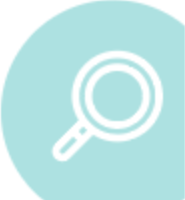
Challenges
Today, claim processing is mostly a manual process involving on-site visits by the insurance company. To validate the farmer's compensation request, insurance representatives visit the affected fields to propose a compensation amount to the farmer. As many farmers might request compensation payments at the same time, this is a lengthy and stressful process for both sides, resulting in a suboptimal client experience and high cost for the insurance company.
Insurance companies need a way to more quickly and easily get the information they need on the damages per field or farmer.
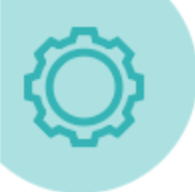
Potential solution approaches
To create a geospatial record of the covered fields, the farmer must specify the fields to be included in the insurance. The field boundaries and corresponding crop types are saved in the insurance companies database as a Shape or GeoTIFF file. Monitoring the crop growth status throughout the year can be automated with optical satellite data (e.g., the freely available data from the Sentinel-2 satellites). A deep learning model like a convolutional neural network (CNN) continuously and automatically analyzes the fields.
In the event of a severe thunderstorm, satellite imagery from after the event can be automatically analyzed, measuring the area and the percentage of crops damaged. Thus, the insurance can provide the farmer with a prediction for the immediate payout.
The immediate payout can later be adjusted, when a more detailed analysis by inspection of satellite imagery or on-site inspections is conducted.
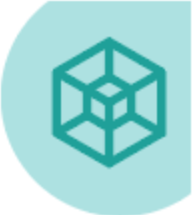