Context
Convective clouds can turn into thunderclouds, which pose a major safety risk to aircraft. Especially in the vicinity of airports, pilots are therefore dependent on reports from meteorologists in order to be able to adjust their flight planning. Detecting such cloud formations or precursors of them reliably and in time requires the full attention of experienced meteorologists.
Automation of cloud detection can thus support meteorologists, save resources, and establish a reliable warning system that reduces the safety risk to aircraft. Existing systems rely on radar data and lightning activity. Analysis of satellite data can provide an additional, independent source for meteorologists.
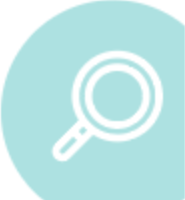
Challenges
Convective cloud detection can be automated with the application of machine learning (ML) methods to meteorological satellite data. By training with known data, an ML model is able to recognize patterns and objects in the imagery and apply what it learns to new data.
However, to do this, the data set must be sufficiently large, and the cloud types must be classified and labeled by hand. Signs of convective cloud formation on satellite imagery are difficult to detect even for well-trained and experienced people. Therefore, labeling the data can be a tedious and error-prone process that definitely needs the supervision of an experienced meteorologist.
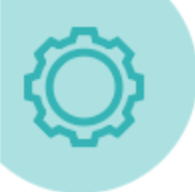
Potential solution approaches
The labeling of the data becomes easier if satellite data in the infrared range are added. This makes characteristics of convective clouds clearer.
There are several algorithms for object detection or image segmentation in image data. The U-Net architecture, which is a special form of a convolutional neural network, has proven itself. This can be used to reliably identify and mark the different cloud types in meteorological satellite data.
The information is made available to meteorologists in real time via an interface.
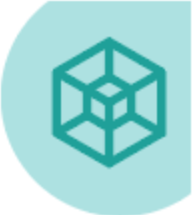