Thatch roofs
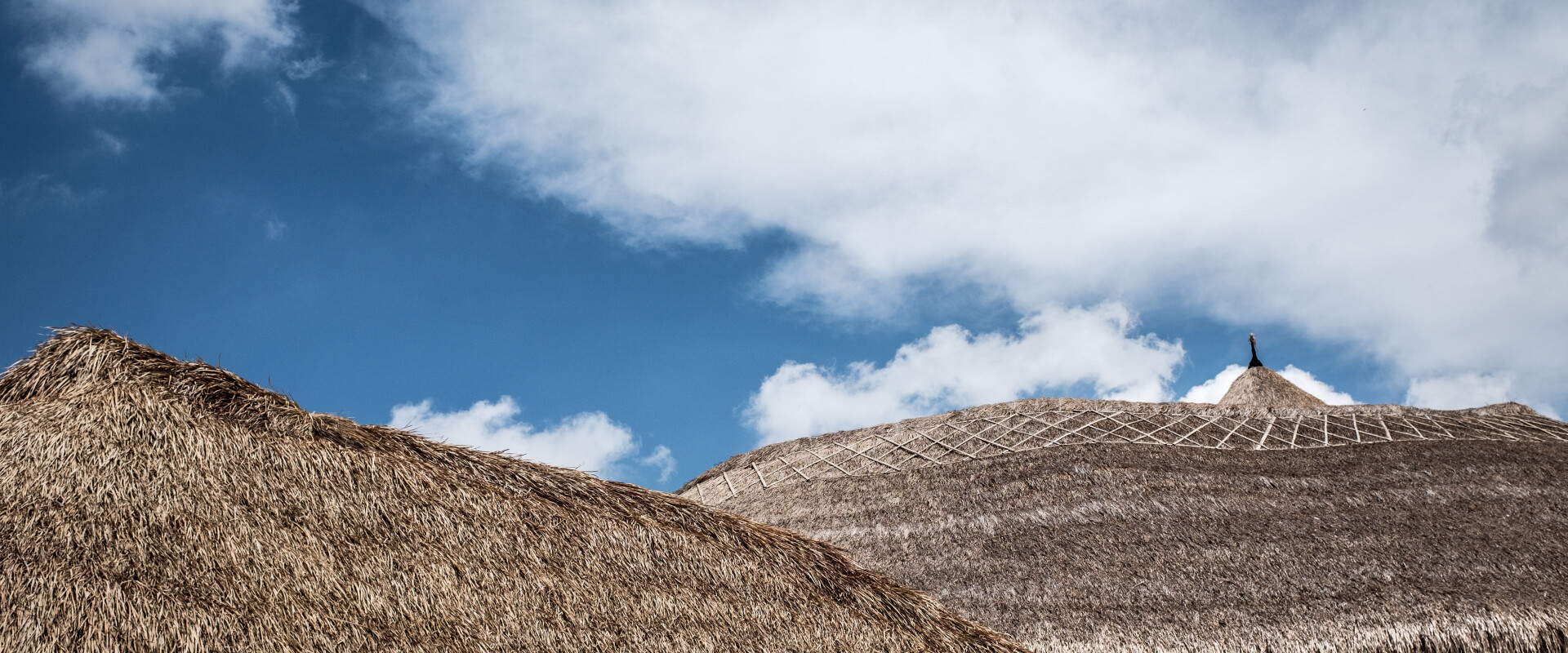
Thatch roof detection for underwriting
Context
The risk assessment of thatch roofs is influenced by many different factors, such as area of the roof, proximity to other roofs, density of roofs in an area, etc. Determining such factors manually and through surveys is a very time-consuming process and can yield inaccurate results.
Automated detection of thatch roofs and determination of areas, distances, etc. can assist the underwriting department. It allows them to provide faster and more accurate risk assessments, which can both increase customer satisfaction and make the underwriting process more efficient.
Challenges
The detection of thatch roofs for risk assessment can be achieved by automatically analyzing satellite images. For this purpose, a machine learning (ML) model can be trained to recognize patterns in satellite imagery based on a labeled dataset and identify a roof as a thatch roof based on that.
The biggest challenge might be to have current image data with a sufficiently high quality available. Open source satellite data, such as ESA's Sentinel data, regularly provide up-to-date data, but could be of too low quality for the detection of thatch roofs. Google Maps data, for example, on the other hand, have a much better resolution, but are not up-to-date for some regions.
Potential solution approaches
The task of object detection and classification in images can be solved by an image segmentation model, for which a convolutional neural network (CNN) is usually used, such as a U-Net architecture. Such a network allows the classification of each image pixel so that roof areas can be distinguished from non-roof areas.
The steepness of the thatch roof can be estimated using projective geometry, since the image data provided are 2-dimensional representations of the roofs. This allows the dimensions and area of the roof to be estimated. Simple metrics can be used to determine the distances to other roofs, or the density of roofs in a previously defined area.