What is defect detection in manufacturing?
dida
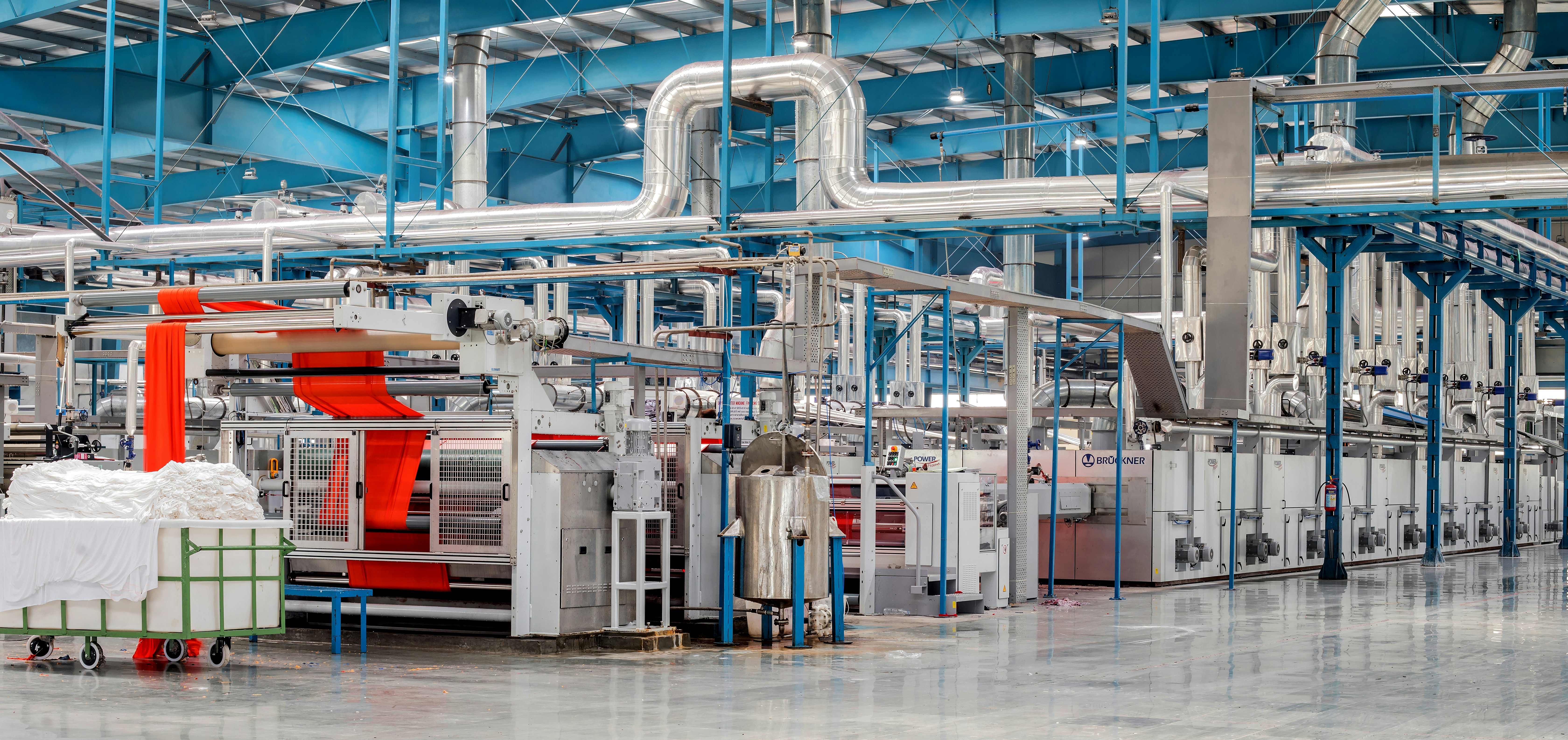
Defect detection is a critical process in manufacturing and quality control, aiming to identify flaws and imperfections in products before they reach the consumer. By ensuring that only high-quality products leave the production line, defect detection systems help maintain brand reputation, reduce waste, and enhance customer satisfaction. This process is essential in various industries, including electronics, automotive, pharmaceuticals, and consumer goods, where precision and reliability are paramount.
Why is it important for quality control?
According to the Systems Sciences Institute at IBM, the cost to fix a defect found post-production can be four to five times higher than one identified during the design phase and up to 100 times more than one identified in the maintenance phase.
Timely detection of defective components is a critical aspect of quality assurance that separates leading manufacturers from mediocre ones. By quickly identifying and correcting defects during the production process, companies can significantly improve their production standards and ensure that only the highest quality products reach the market. This proactive approach not only saves money, but also improves customer satisfaction and brand loyalty. As a result, manufacturers who invest in advanced defect detection technologies set themselves apart as industry leaders committed to excellence and reliability. The higher the degree of automation for detecting defects in contrast to purely manual inspections, the cheaper and often more reliable the process is.
How to identify defects in the production line?
Identifying defects in products involves a systematic approach that combines various inspection methods and technologies. Visual inspection, often the first step, employs trained personnel or machine vision systems to spot obvious flaws. Non-destructive testing methods, such as ultrasonic, magnetic, and radiographic inspections, delve deeper to uncover hidden defects without damaging the product. Automated systems enhanced with artificial intelligence and machine learning algorithms can detect subtle and complex defects by analyzing data patterns. Additionally, statistical quality control techniques, including sampling and control charts, help in identifying trends and anomalies in production processes. In the following we will have a closer look at these techniques and explain which one is better for which approach.
What are defect detection techniques?
1. Visual inspection system:
Visual inspection is one of the most common methods used to catch defects in products. It involves manual inspection by human eye, automated anomaly detection by cameras, or artificial intelligence systems. Human inspectors rely on their trained eyes to detect irregularities such as cracks, scratches, or misalignments that could compromise product quality. Alternatively, cameras equipped with vision software or AI algorithms can automate this process, improving speed and consistency. However, without AI systems included, visual inspection has its limitations; it can miss defects that are subtle or located in hard-to-reach areas. In addition, human inspectors can suffer from fatigue and variability in judgment over time, affecting the reliability of the inspection process. Despite these challenges, visual inspection remains invaluable in quality control due to its accessibility, versatility, and ability to efficiently detect visible defects.
2. Non-destructive testing (NDT):
Non-destructive testing (NDT) is a method that examines the structure and properties of a product without altering its integrity. It utilizes physical phenomena like sound, light, magnetism, electricity, or radiation to probe for defects that may be hidden or inaccessible to visual inspection. Moreover, NDT can measure various characteristics such as thickness, hardness, density, and composition, providing comprehensive insights into product quality and performance. This versatility makes NDT indispensable in industries where precision and reliability are paramount, ensuring that products meet stringent quality standards before reaching the market.
3. Statistical process control (SPC):
Statistical Process Control (SPC) uses statistical tools and techniques to monitor and manage quality and variability in manufacturing processes. By analyzing data over time, SPC identifies abnormal or unstable conditions that can lead to defects, such as shifts, trends, cycles or outliers. This proactive approach allows manufacturers to take timely action to ensure consistent product quality and minimize the risk of defects reaching the customer. Take a look at one of our AI projects where we optimized machinery parameters in order to optimize production outputs.
4. Design of experiments (DOE):
Design of experiments (DOE) is useful for researchers and engineers who want to systematically optimize processes and improve product quality. Through the methodical design and execution of experiments, DOE helps identify key factors affecting a process or product and their interactions. This approach allows for the efficient exploration of multiple variables under controlled conditions, leading to insights that can improve performance, reliability, and efficiency. DOE is particularly valuable in industries such as manufacturing, engineering, and pharmaceuticals, where precise control of variables is critical to achieving desired results and minimizing errors.
5. Machine learning and computer vision
Machine learning, with computer vision as a critical subcomponent, has innovated defect detection by using advanced algorithms to analyze massive amounts of data. These algorithms can identify subtle patterns that indicate product defects.
By training neural networks on annotated datasets, computer vision systems can detect anomalies that traditional methods might miss, improving accuracy and efficiency in industries such as manufacturing, electronics, and automotive. These algorithms continuously evolve with new data, minimizing false positives and improving overall product quality assurance. Integrating computer vision into machine learning frameworks streamlines inspections, increases productivity, and ensures higher customer satisfaction by providing robust and accurate defect detection.
Defect detection at dida
At dida, we’re defect detection specialists, having worked on many defect detection projects in the past. Here you can find a selection:
One example is our project on defect detection in manufacturing, where we detected errors in the production of semiconductor lasers.
In another project, we developed an AI solution for defect detection in 3D printing and additive manufacturing
We also published this scientific defect detection paper together with some of our partners.
No matter the method, effective defect detection hinges on robust data collection; when this data collection meets the need for precise judgement, the choice usually leads to machine learning.
For more information about dida or defect detection, we recommend you to visit our website to learn more and take the next step towards flawless production.