Stock trading
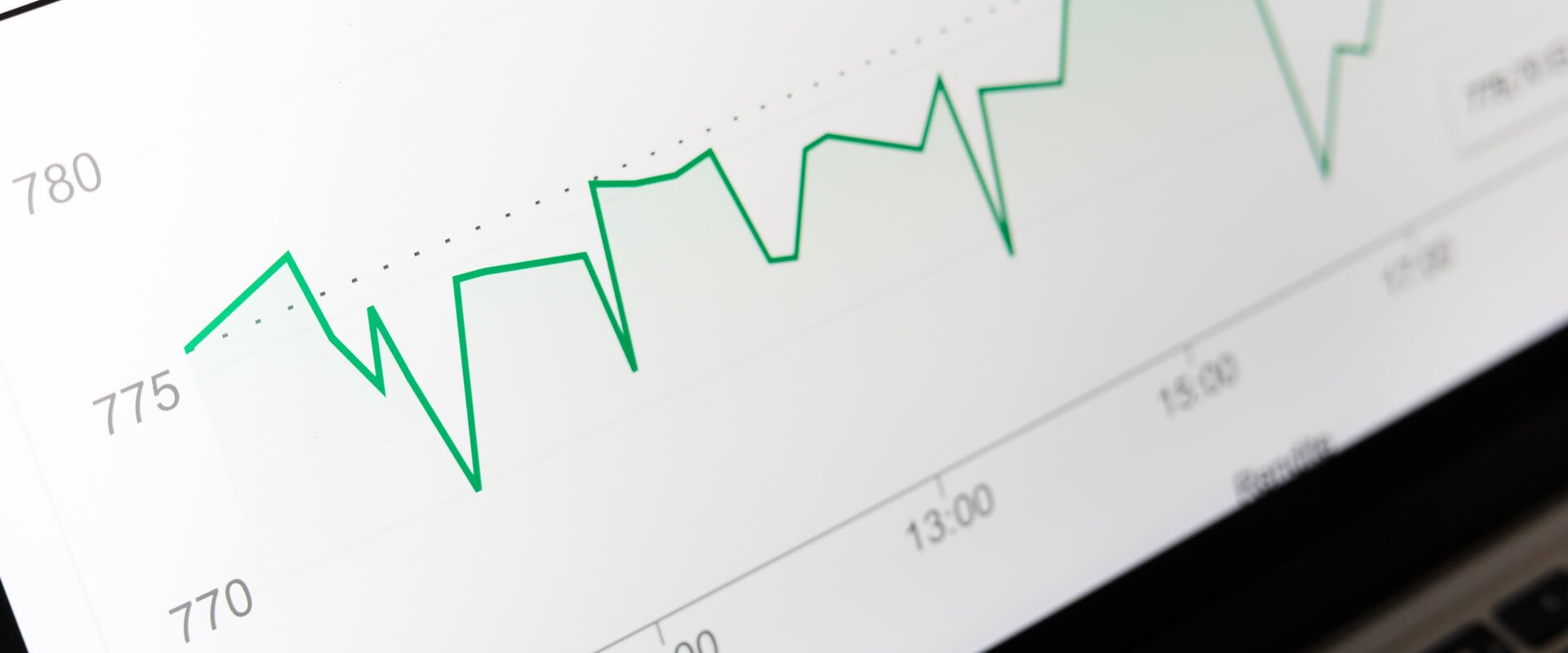
Stock trading based on sentiment analysis
Context
Share prices of public companies are influenced by bank analysts evaluating a company's financials and investor relation communications. Analysts and journalists are constantly publishing articles and reports to evaluate the company's performance in the market space and comparing it to competitors. After the quarterly earnings report, analysts and journalists evaluate if the company's stock price is still reasonable. It might be under- or overvalued if the company did not meet market expectations or exceeded them.
Challenges
When companies publish ad-hoc statements or financial statements, (potential) investors are investigating articles and analysts' reports to conclude if there is an arbitrage opportunity, i.e. if the stock is under- or overvalued. However, apparent arbitrage opportunities vanish quickly as professional stock traders react within a short period of time to generate profits. Evaluating the effect of news on stock prices in a matter of seconds or even quicker is therefore essential to capitalize on arbitrage opportunities.
Potential solution approaches
Sentiment analysis is a machine learning technique to classify text into different classes of sentiments the author carried while writing the text. The most simple form is a classification into 'positive', 'negative' or 'neutral'. More sophisticated algorithms classify the emotional state (e.g. 'angry') or the magnitude of sentiment (e.g. on a scale from 1 to 5). Algorithms used for sentiment analysis include naive Bayes regression and support vector machines (SVM). For more sophisticated tasks, deep neural networks can be suitable.