Banking
Use cases: Machine Learning Solutions
The banking and finance industry is a prime candidate for optimization via Machine Learning methods due to the quantitative nature of the sector. Financial transactions generate enormous amounts of numerical data, which can then be fed to Machine Learning models. By analyzing this data, they learn typical patterns, even those unrecognizable to humans. They are also suited for helping with more robust security or reducing the workload of administrative personnel.
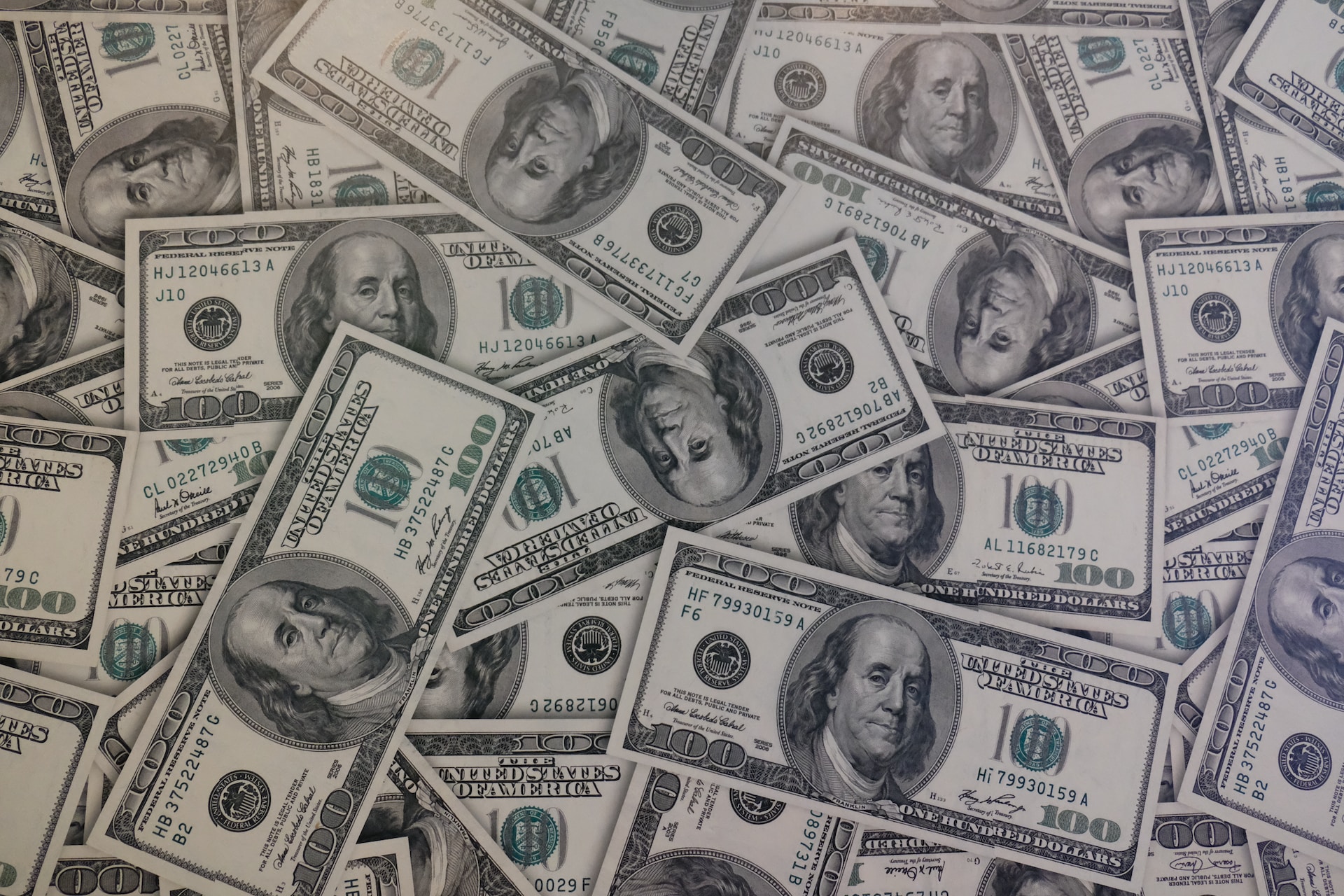
Improving compliance operations e.g. by backing Anti-Money Laundering (AML) surveillance with Machine Learning algorithms
A Machine Learning model trained to detect anomalies can learn complex transaction patterns using historical data and find which current transactions deviate from those patterns. Even with the money laundering schemes changing over time, the system can keep itself up-to-date by including any new patterns it learns. The models are also precise enough to also reduce the number of False Positives.
.jpg)
Capitalizing on arbitrage opportunities through accelerated information processing
In order to take advantage of arbitrage opportunities, constant monitoring and a thorough understanding of the fluctuations of all relevant markets is needed and an above average accuracy is crucial. A time series analysis based on Machine Learning models can quickly analyze historical data and forecast probable future scenarios in order to maximize gain and minimize risk of loss.
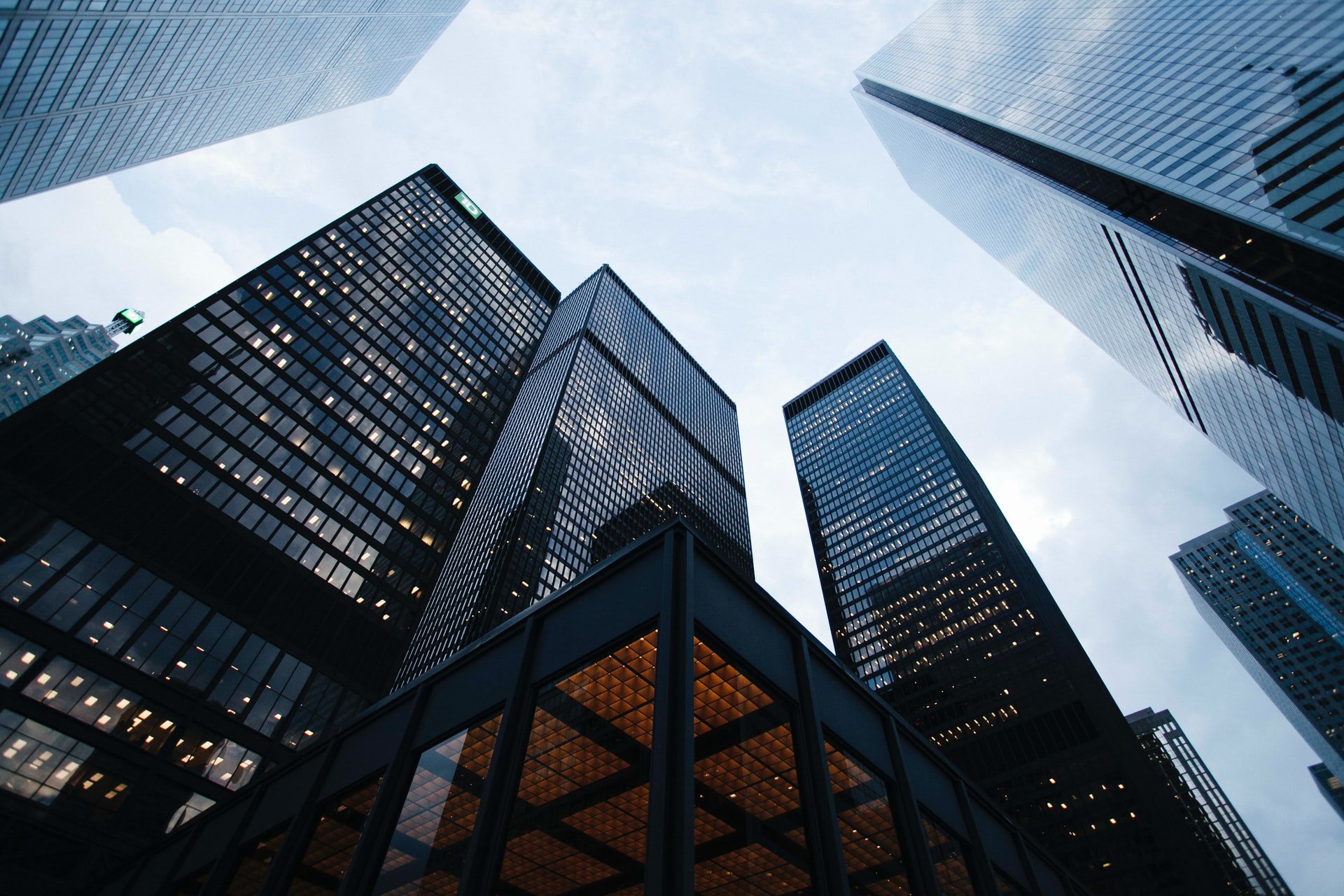
Automating the information extraction of key metrics from annual reports of publicly listed corporations
Keeping informed about publicly listed corporations’ activities is a time-consuming process needing human experts. An Information Extraction system can reduce the workload of human employees by automatically detecting the important pieces of information from the annual reports.