A bitcoin on top of a dollar bill
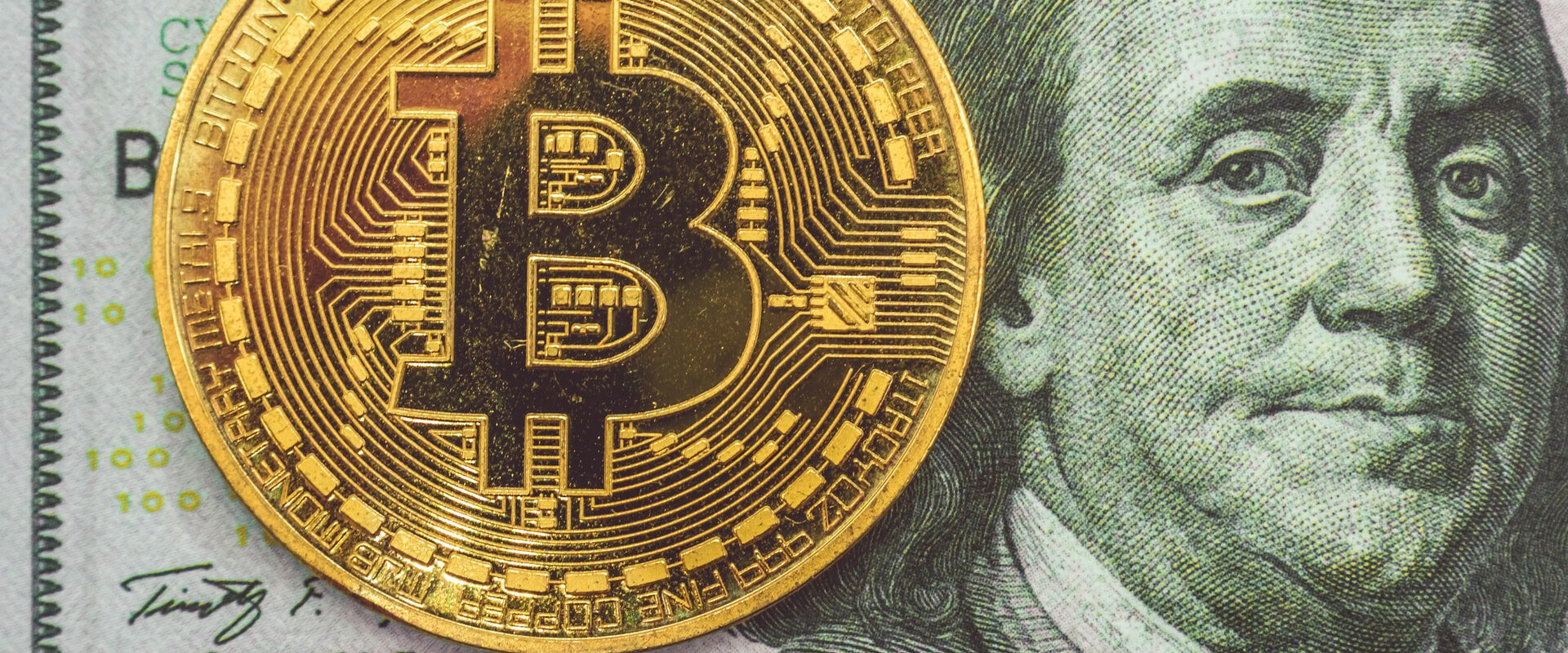
Anti-money laundering (AML)
Context
According to the United Nations Office on Drugs and Crimes (UNODC) between 800 billion to 2 trillion US dollars from criminal activities are laundered per year, representing two to five per cent of the world's gross domestic product. A money laundering scandal at Danske Bank revealed in 2018 involved more than 229 billion USD and the bank and their executives have been made accountable for not acting on earlier indications from whistleblowers.
Challenges
Banks are increasingly made responsible by authorities for not establishing appropriate control mechanisms to prevent money laundering through the company's bank accounts. At the same time, Bitcoin transactions become increasingly popular among criminal organisations due to their pseudonymity.
Traditional rule-based approaches have led to a large number of "false positives" which have to be processed manually and with highest care. If bank accounts are closed due to a false positive classification, clients are upset and the bank risks to lose business with this client. From a technical perspective, it might be difficult to access and obtain labeled datasets for supervised learning approaches due to high data security barriers.
Moreover, synthetic or augmented data might not represent the real distribution of fraudulent transactions, as money laundering tactics are diverse, change over time and result in dynamic data.
Potential solution approaches
Rule-based approaches of fraudulent transactions might still be a good starting point if certain patterns can be identified with certainty from observation. For example, transactions from tax havens, such as the Cayman Islands, might have a higher probability of being fraudulent.
Algorithms used regularly for anomaly detection include k-nearest neighbours, support vector machines or Bayesian networks. More sophisticated machine learning approaches might be chosen to detect less obvious patterns, especially in the case of a limited amount of training data or dynamic data.
Graph neural networks can map hierarchical relationships, e.g. between entities, countries or banks and therefore make transaction patterns easier to compare. Active learning might help to create labels by reviewing suspicious patterns from the model output and constantly track and include labels over time.