© unsplash@thisisengineering
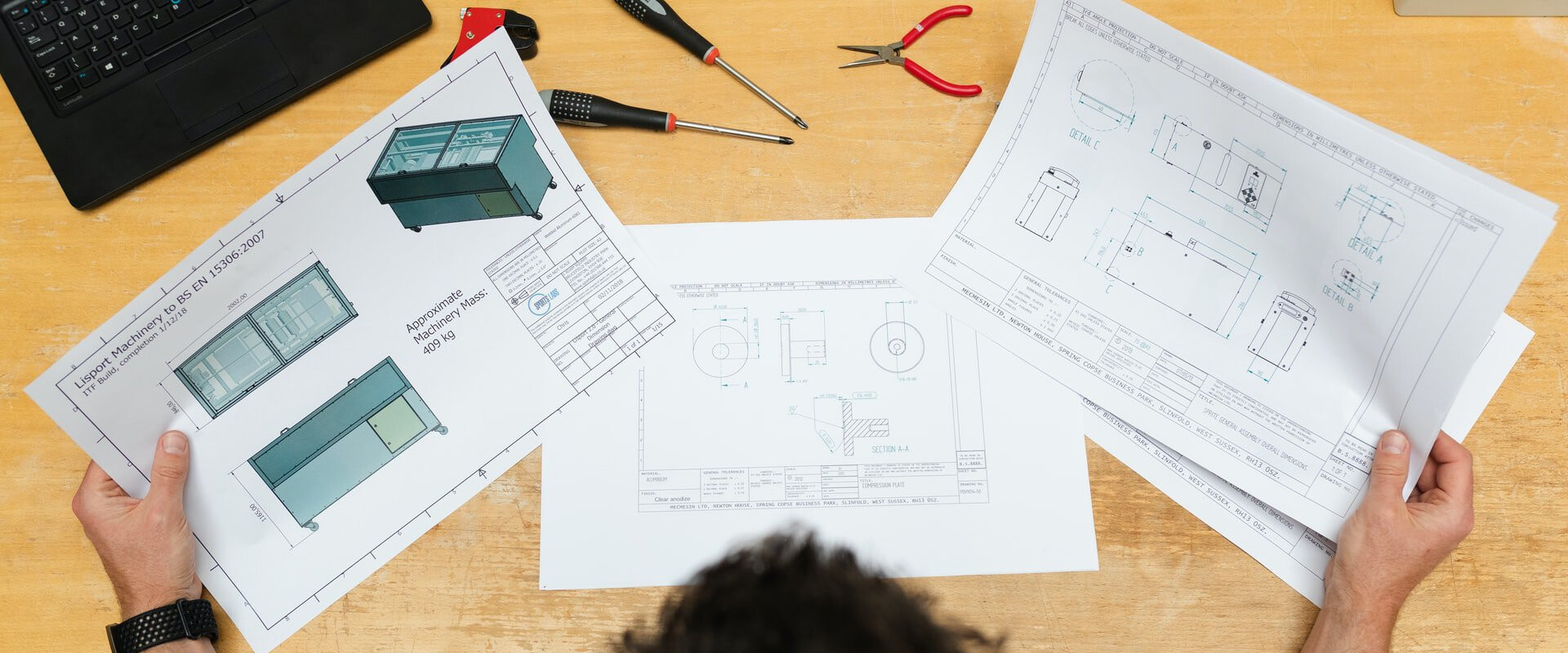
Information extraction from technical manuals
Context
Technical manuals provide the basis for technicians to maintain production plans, cars or virtually every type of machinery. For engineers and mechanics, it is often difficult to find the relevant information for the task at hand, as most manuals or technical drawings are either paper-based or pdf files. If the files are available as pdf, it remains a challenge to find relevant information as manuals often have tens or hundreds of pages and searching within documents is keyword based without further semantic understanding.
Challenges
Most mechanical work implies working with machines from different manufacturers. As there is no uniform format and terminology across manufacturers, it is often challenging to find the same type of information for models of different manufacturers. Moreover, even for one manufacturer, format and terminology might change over time, especially if the machinery is long-lived.
Another challenge is that content within manuals come in very different formats such as tabular data, free text, bullets lists or drawings. Since the information mechanics are looking for is often found in a combination of these formats, a solution solely based on NLP algorithms might not deliver satisfactory results. Moreover, training data might be scarce as some parts might be prevalent only in a small fraction of models.
Potential solution approaches
Imagine the focus lies on the NLP part of the project, it is an option to train a model based on generic contextualized word embeddings such as BERT. BERT has the advantage of including synonyms and context within free text and strings compared to traditional word embeddings such as Word2Vec and fasttext and is state-of-the-art in modern NLP applications. However, it could be shown that for very specific domains such as engineering, domain specific word embeddings might increase the model performance.
A (domain specific) BERT embedding can then trained to perform question answering, a technique to allow mechanics to ask natural language questions to receive highlighted areas of the manual concerning this particular tasks.