Back Office
Use cases: Machine Learning Solutions
Back office tasks are often monotonous and tend to involve processes where a small oversight can cause larger problems. Fortunately, they are also often a textbook case for being supported by automation. Machine Learning models never lose focus and precision and excel at dealing with large quantities of data. They also have scalability benefits: they remain efficient even if the number of incoming tasks grows significantly.
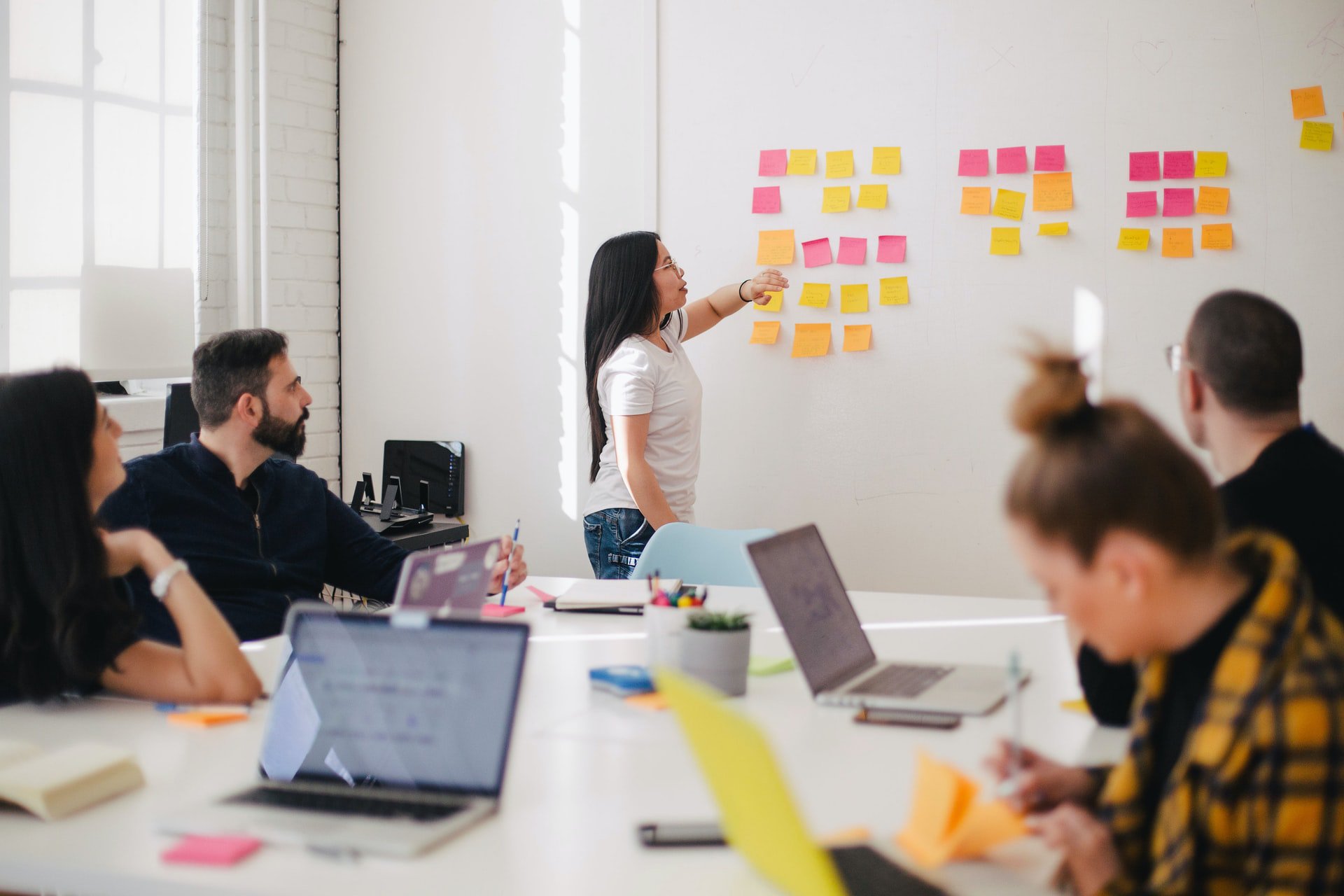
Saving time and resources in non-value creating tasks, e.g. by assigning incoming documents or requests automatically
Companies receive a large number of documents daily, often including personal or sensitive data. While manually determining how to proceed dealing with them is time-consuming, an Optimal Character Recognition system can digitize handwritten texts for easier further processing and Information Extraction models can find the key pieces of information in order to automatically classify these documents.
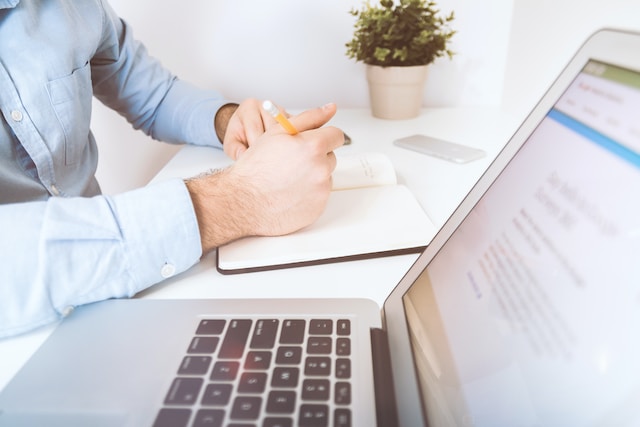
Helping employees and customers find relevant information to their request easily and without additional human interaction
A Question-Answering system built on Natural Language Processing models can find answers or guide people to the relevant source. Using an automated system as a first step can cut back on the employee’s time so they can focus on more complex queries. An additional benefit is that the system can update itself, so the next time a similar question is asked, it will already know the correct answer.
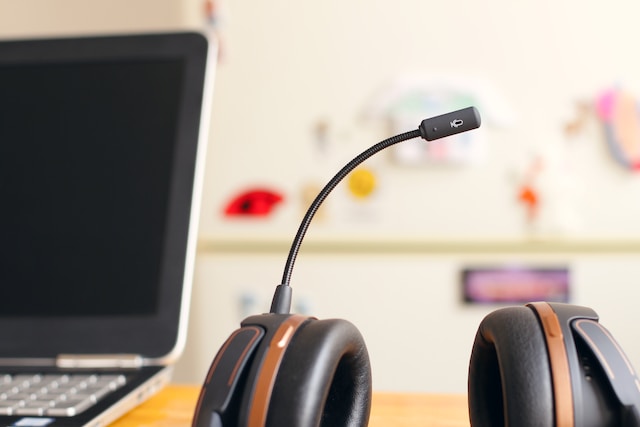
Reducing time lapse between request and answer and increasing customer service quality
Introducing a chat bot can improve customer experience. Oftentimes questions can quickly be answered by the bot itself with no waiting time. If that is not the case, the bot can label the questions and connect them to the customer’s profile or order by the time the human customer service representative enters the chat.