Context
The production volume of mining activities must be quantified on a regular basis in order to be able to make financial and logistical plans and meet regulatory requirements, for example for the calculation of the production index. It is essential to have access to fast and validated estimates.
In mining, mined minerals and ores are collected and stored in large stockpiles. Automatic calculation of the volume of such stockpiles can be an efficient method for fast and reliable quantification of the production volume.
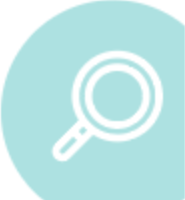
Challenges
The calculation of the volume of stockpiles can be automated by a machine learning (ML) model that analyzes image and radar data from the stockpile and creates a 3D model that can be used to calculate the volume.
The main challenge here is to find a data source that provides high-resolution image and radar data at short time intervals to obtain the most accurate calculations at regular and steady time intervals. Either photogammetric threat data or high-resolution satellite data can be used for this purpose. However, open source satellite data, such as those from ESA's Sentinel missions, are less suitable for stockpile volume analysis due to the above requirements.
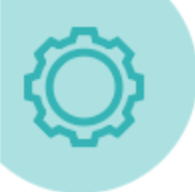
Potential solution approaches
Automatic analysis of image and radar data can be achieved by modern ML and Computer Vision (CV) algorithms, such as Convolutional Neural Networks (CNN). These are able to detect and classify objects in images by training with a sufficiently large labeled data set.
This allows delineations, ground level, elevation profiles, and other geometric properties of stockpiles to be determined in the image and radar data, and a digital elevation model to be created. The volume calculation is then performed using common geometric methods, such as the cross-section method or by the method via prisms between two surfaces.
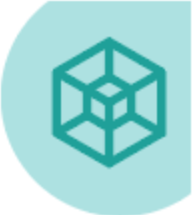