Power poles affected by a power outage
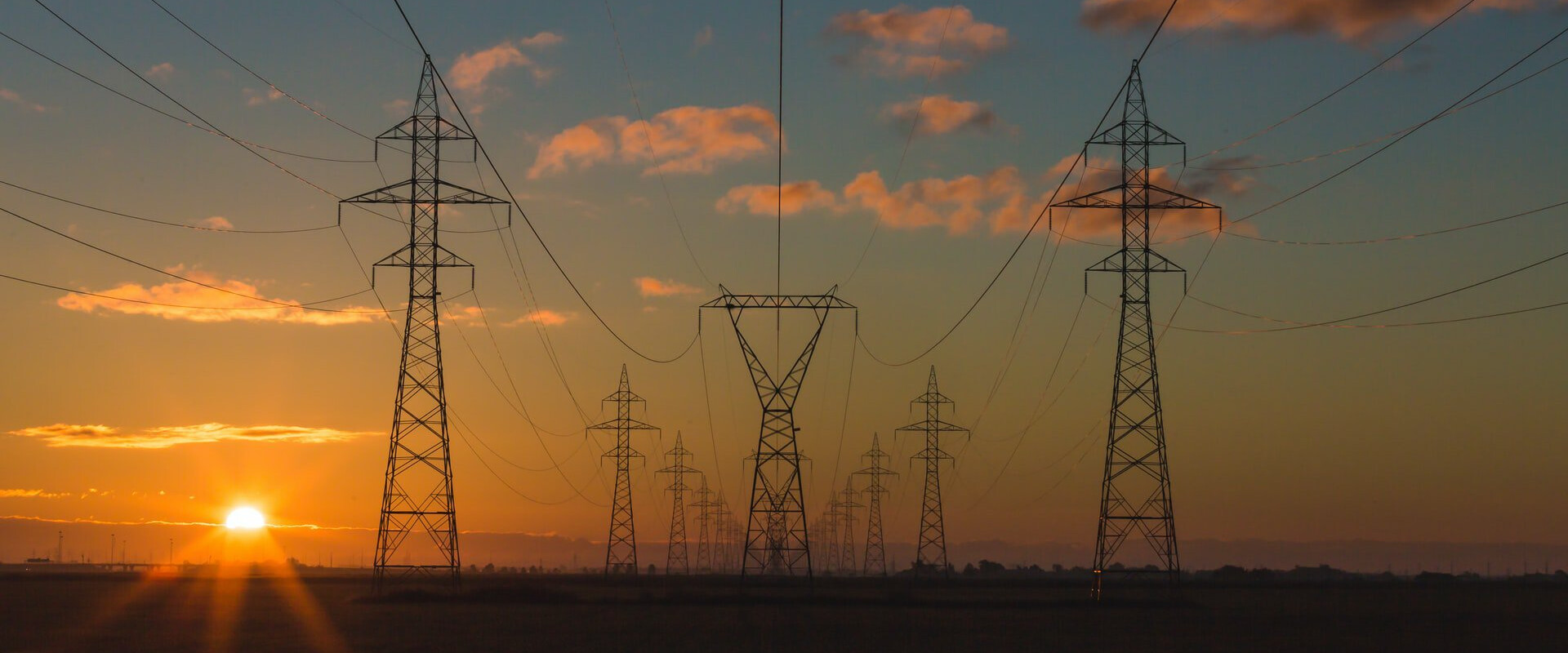
Power outage prediction
Context
In 2019, every inhabitant of Berlin was without electricity for an average of 34 minutes, which was a new negative record. The economic loss caused by production stops during the outage easily surpasses millions of Euros and may lead to legal proceedings on the liability.
Challenges
Power outages are often connected to weather conditions, outdated infrastructure components, congested networks or on a change of vegetation and landscape. Therefore, to produce a dataset that covers all relevant features, information on past outages must be combined with weather, infrastructure, and earth observation data.
Potential solution approaches
Suitable earth observation data are satellite images in the radar and visible spectrum. Artificial neural networks (e.g., Convolutional Neural Networks) can recognize and classify objects on these images. This can be used to detect changes in vegetation and the landscape over time; for example, trees near power lines can be monitored.
For a reliable forecast model, the different data sources have to be combined. To process the features from the vegetation assessments and weather forecast data, models such as Decision Trees or Regression Trees (e.g. BART) are suitable. The training of the combined model is done using the historical failure dataset. The trained model is then able to predict from current data where the probability of failures is highest.