Infrastructure from the power net
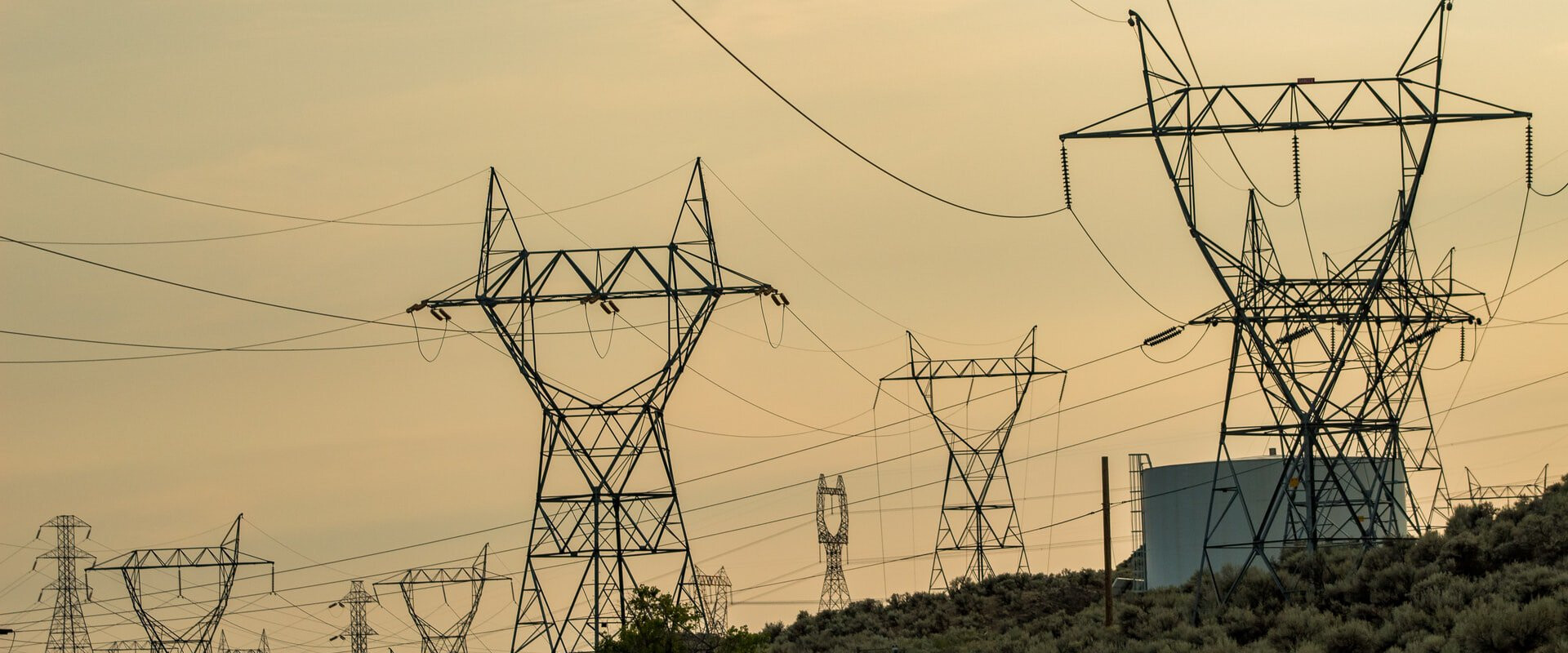
Infrastructure monitoring
Context
Companies in the utility sector have large infrastructure networks such as gas pipeline or electricity grids to ensure a comprehensive supply. The monitoring of this network is essential because it provides information for maintenance, outages and possible supply gaps and therefore relevant for security, especially in densely populated areas. However, it is difficult to receive information about maintenance needs prior to the actual damage and the inspection and monitoring of infrastructure networks on the ground is very laborious and time-consuming.
The use of satellite and UAV (unmanned aerial vehicle) data in the required spatial resolution and their automated analysis might enable utility corporations to monitor more efficiently as anomalies can be detected earlier and early warnings might be generated automatically.
Using an AI-driven satellite monitoring system, the Deutsche Bahn for example were able to reduce the storm damage caused by trees by 25 %.
Challenges
The automated monitoring of infrastructure networks requires the real-time processing of satellite data to provide information about the condition of the network in high time intervals. Depending on the satellite data used, new satellite data is created within 3 - 15 days. The automated data processing can be accomplished by programming a machine learning model, which is able to recognize patterns and anomalies in satellite imagery, e.g. construction work close to gas pipelines.
Satellites can provide image data in different wavelength regimes, e.g. in the visible and radar spectrum, which contain different information about the observed territory. As an example, Sentinel-1 radar data provides information about vegetation and water among others, whereas Sentinel-2 data carries information in the visible spectrum (RGB).
Potential solution approaches
For processing satellite data, the machine learning algorithm needs to be capable of detecting patterns, i.e. objects such as construction work or changes in the surface, in the satellite image or radar data and to classify them. This can be accomplished by employing a model for image segmentation, which is commonly a convolutional neural network (CNN).
The information extracted from the data has to be compared to previous image information to identify anomalies.
The derived information can also be used as a data basis for a time series forecasting model to predict outages. Moreover, the model can be combined with IoT data generated along the pipeline or grid, if available.