Context
Over the course of human history we chopped down large areas of forests and are continuing to do so, just to discover its severe consequences like desertification more and more. In an effort to prevent furure damage and halt advancing deserts, there is ever growing interest in reforestation like the public great green wall in china or private impact investment projects.
But since the early days of regreening we have learned that the job is not done with planting the trees: one has to continually check the health of the young forest areas to act fast in case of destabilization until a strong and diverse ecosystem is reestablished.
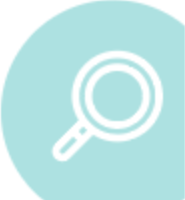
Challenges
Here in densely populated Germany we have the luxury to employ 'Förster'. These are people who continuously work in and with every small forest and therefore know the health of the trees very well.
In large reforestation projects this is too expensive to do. Even obtaining drone footage can be very costly, as reforested areas are often remote and can span large areas. Moreover drone images lack the consistency of satellite observations.
Still, after obtaining images for the region of interest someone has to go over all these pictures and figure out the health of the plants. This is a labour-intense process and often only encompasses coarse analysis like looking at the 'greenness'. Therefore small changes are easily overlooked by the human eye. Also one can only have a look at so many datasets at once, making it difficult to relate between them and over time.
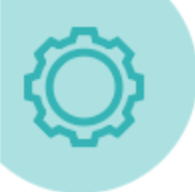
Potential solution approaches
A more sophisticated approach is to use hyperspectral satellite imagery: here more wavelengths are used than the 3 colors our eyes can perceive, therefore we can obtain much more information about the things we look at. Those additional bands enable us to calculate 'new colors' like the normalized difference vegetation index (NDVI) to discover vegetation or the solar-induced chlorophyll fluorescence (SIF) index to see how photosynthetically active this vegetation is.
To condense all the information and combine recent observations with past ones, AI approaches like neural networks (NN) with temporal encoding are a good choice. They offer new ways to spot illness patterns early and discover weaknesses in the ecosystem before the issues jeopardize the success of long replanting efforts.
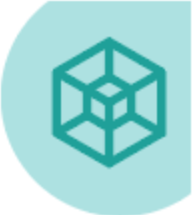