Context
Ensuring the yield of crops and the quality of agricultural land requires a fast detection of pest and weed infestation to prevent an extensive infestation and to use targeted pesticides, e.g. by precision farming techniques.
Also regarding climate change and corresponding effects, such as the changing flora, fauna and soil conditions, an automated detection system can help farmers to use eco-friendly and efficient control methods.
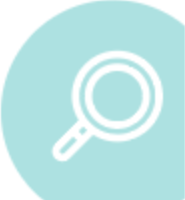
Challenges
The monitoring of agricultural land and the automated detection of pest and weed infestation can be solved by using a machine learning model, that processes satellite and UAV imagery, which are used for real-time and automated monitoring of agricultural areas providing data in the visible and radar spectrum. Such a model is able to recognize patterns in the image data, such as changes in colour and shape of the acres caused by weed and pest infestation, and classifies the different objects by their vegetation or infestation type.
However, in order to achieve accurate and reliable results, a large data set is required to cover all important infestation types in different stadiums, which remains the major challenge for the automated detection of weed and pest infestation. UAV or drone imagery is also very costly to acquire, at least in the coming years, so that automated field management and precision farming with drones will not be viable in the coming years.
Satellite imagery, however, at least open access satellite imagery, does not have the required spatial resolution to detect infestations at an early stage and might be only be feasible at later stages of the infestation.
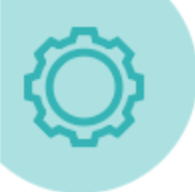
Potential solution approaches
Detecting pest and weed infestation requires a machine learning algorithm for image segmentation and classification in order to detect and classify different objects and patterns in the image data. Such computer vision tasks are commonly solved by special convolutional neural networks (CNN), such as U-Net or Mask R-CNN architectures.
These are able to detect differences in color and shape of the agricultural areas and can identify infested areas on the basis of labeled training data as well as the infestation types based on their influence on the crops and vegetation.
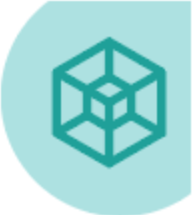