Paper on solving parabolic and elliptic PDEs with deep learning
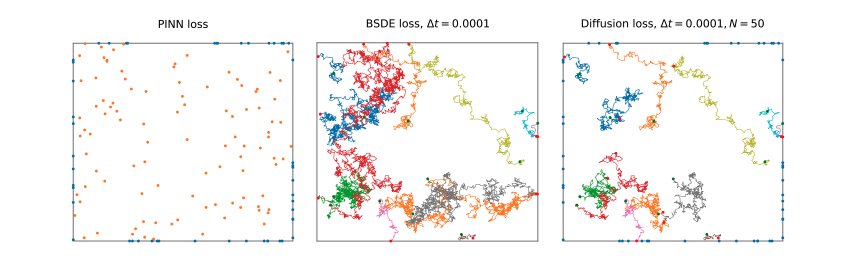
Let us refer to the a new research paper on a machine learning attempt for solving high-dimensional PDEs that has recently appeared on Arxiv and has been done in collaboration with dida. In the paper, we analyze existing algorithms that are based on residual minimizations („physics informed neural networks“) or backward stochastic differential equations („deep BSDE“) and suggest a new method that is based on the novel diffusion loss. Crucially, this method can be interpreted as an interpolation between the two existing methods that, at the same time, offers certain numerical advantages. Those advantages may allow for more efficient and more accurate PDE approximations and are therefore likely to find further applications whenever high-dimensional PDEs have to be solved.