Airport with runways
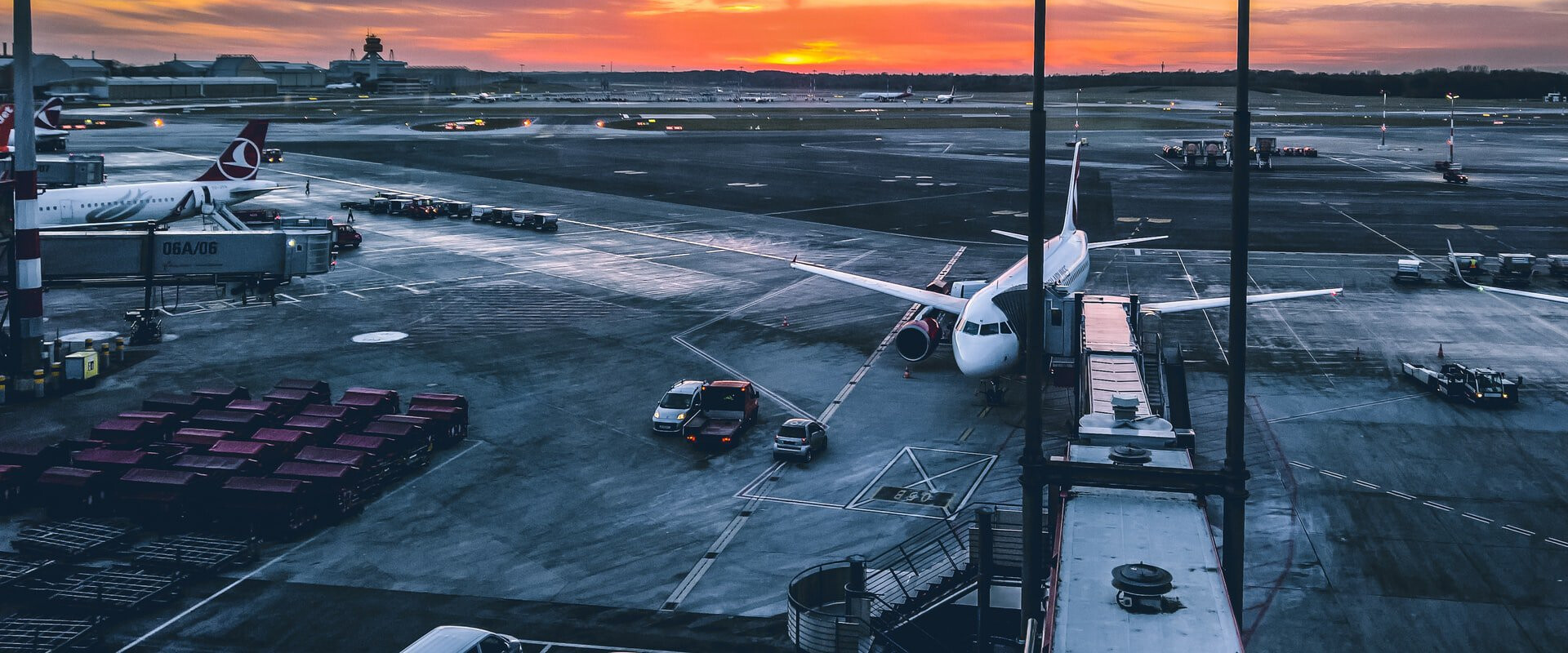
Automatic object detection on airport runways
Context
Airport runways must be constantly controlled and monitored by air traffic control to detect safety-critical objects such as flocks of birds or vehicles. This process requires constant attention and focused work by the air traffic controllers to ensure safe take-offs and landings. Automating object detection can help controllers identify potential risks on the runway.
Challenges
The automatic object detection on airport runways requires the processing of image and video data in order to detect and classify objects such as birds, airport staff, or baggage cars. This can be done by a machine learning model which recognizes patterns in image data based on shapes and colours.
The greatest challenge is to generate a training data set that provides sufficiently labelled data of good quality. It should cover all conceivable objects on runways in order to reliably detect them during operation.
In addition, the system requires a very high level of accuracy. In no case should dangerous objects be overlooked, but there should also not be a high number of "false positives" in order not to weaken the confidence of the air traffic controllers in the software.
Potential solution approaches
An image segmentation and classification model is suitable for detecting and classifying objects in images and videos. State of the art algorithms are Convolutional Neural Networks (CNN) such as U-Net or Mask-R-CNN architectures.
To reduce the required amount of training data and therefore cost, pre-trained models such as VGG-16 or ResNet50 can be used. For video data, a YOLO ('You only look once') architecture might be suitable.
While the object detection part might be relatively straightforward to implement, the major effort is in assessing situations in terms of flight safety. For example, a flock of birds flying toward the runway is more dangerous than a flock flying away from the runway.
A vehicle crossing a runway is less critical if prior permission was given by air traffic control. Handling exceptions and incorporating air traffic control knowledge is therefore essential to be able to use the software in production.