Interpretable Neural Networks with Frank-Wolfe: Sparse Relevance Maps and Relevance Orderings
by Jan Macdonald, Mathieu Besançon, Sebastian Pokutta
Year:
2022
Publication:
Interpretable Neural Networks with Frank-Wolfe: Sparse Relevance Maps and Relevance Orderings
Abstract:
We study the effects of constrained optimization formulations and Frank-Wolfe algorithms for obtaining interpretable neural network predictions. Reformulating the Rate-Distortion Explanations (RDE) method for relevance attribution as a constrained optimization problem provides precise control over the sparsity of relevance maps.
Link:
Read the paperAdditional Information
Brief introduction of the dida co-author(s) and relevance for dida's ML developments.
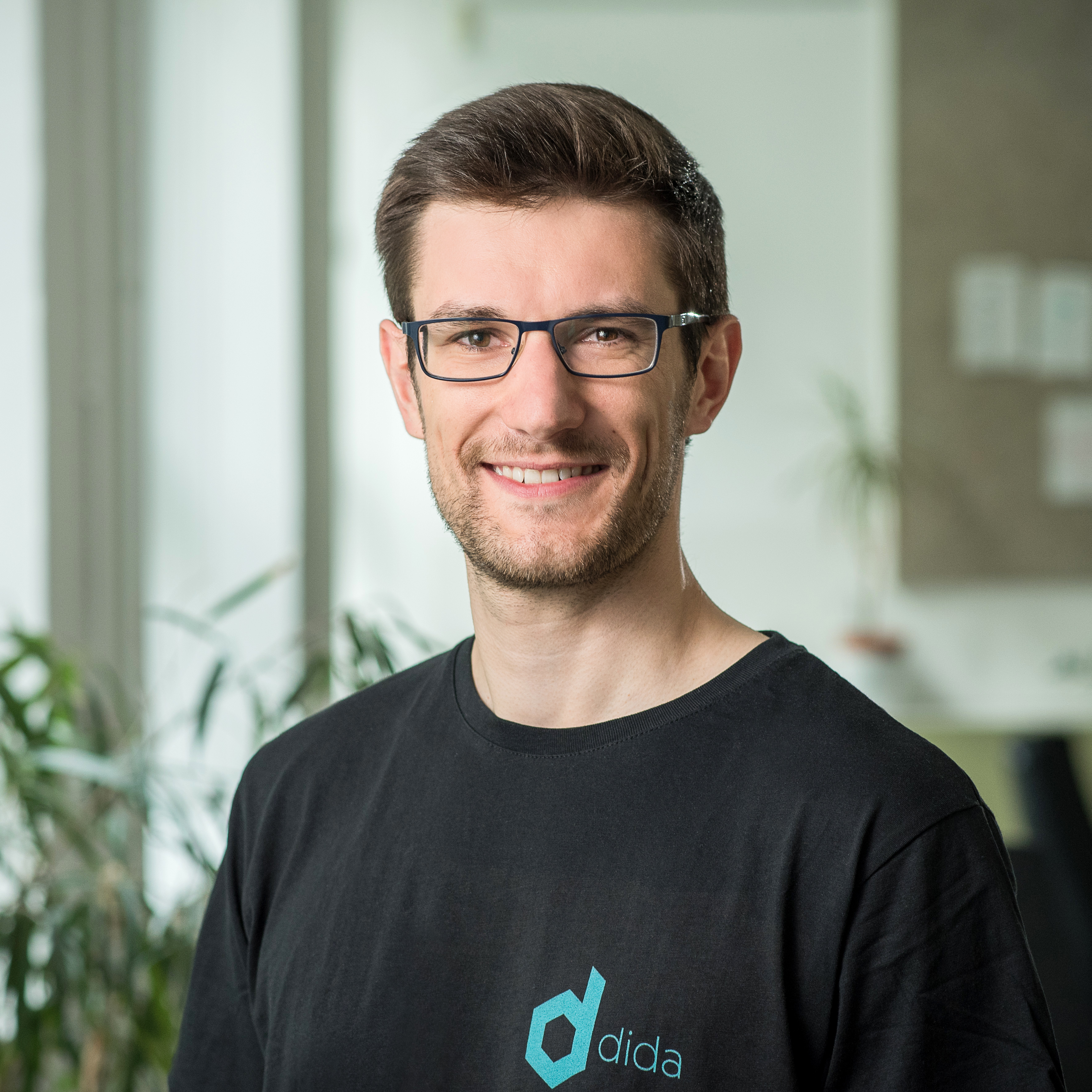
About the Co-Author
During his studies in mathematics (TU Berlin) Jan focussed on applied topics in optimization, functional analysis, and image processing. His doctoral studies (TU Berlin) explored the interplay between theoretical and empirical research on neural networks. This resulted in his PhD thesis investigating the reliability of deep learning for imaging and computer vision tasks in terms of interpretability, robustness, and accuracy. At dida he works as a Machine Learning Researcher at the interface of scientific research and software development.