Let's Enhance: A Deep Learning Approach to Extreme Deblurring of Text Images
by Theophil Trippe, Martin Genzel, Jan Macdonald, Maximilian März
Year:
2023
Publication:
Inverse Problems and Imaging, 17(5): 1041-1068.
Abstract:
This work presents a novel deep-learning-based pipeline for the inverse problem of image deblurring, leveraging augmentation and pre-training with synthetic data. Our results build on our winning submission to the recent Helsinki Deblur Challenge 2021, whose goal was to explore the limits of state-of-the-art deblurring algorithms in a real-world data setting.
Link:
Read the paperAdditional Information
Brief introduction of the dida co-author(s) and relevance for dida's ML developments.
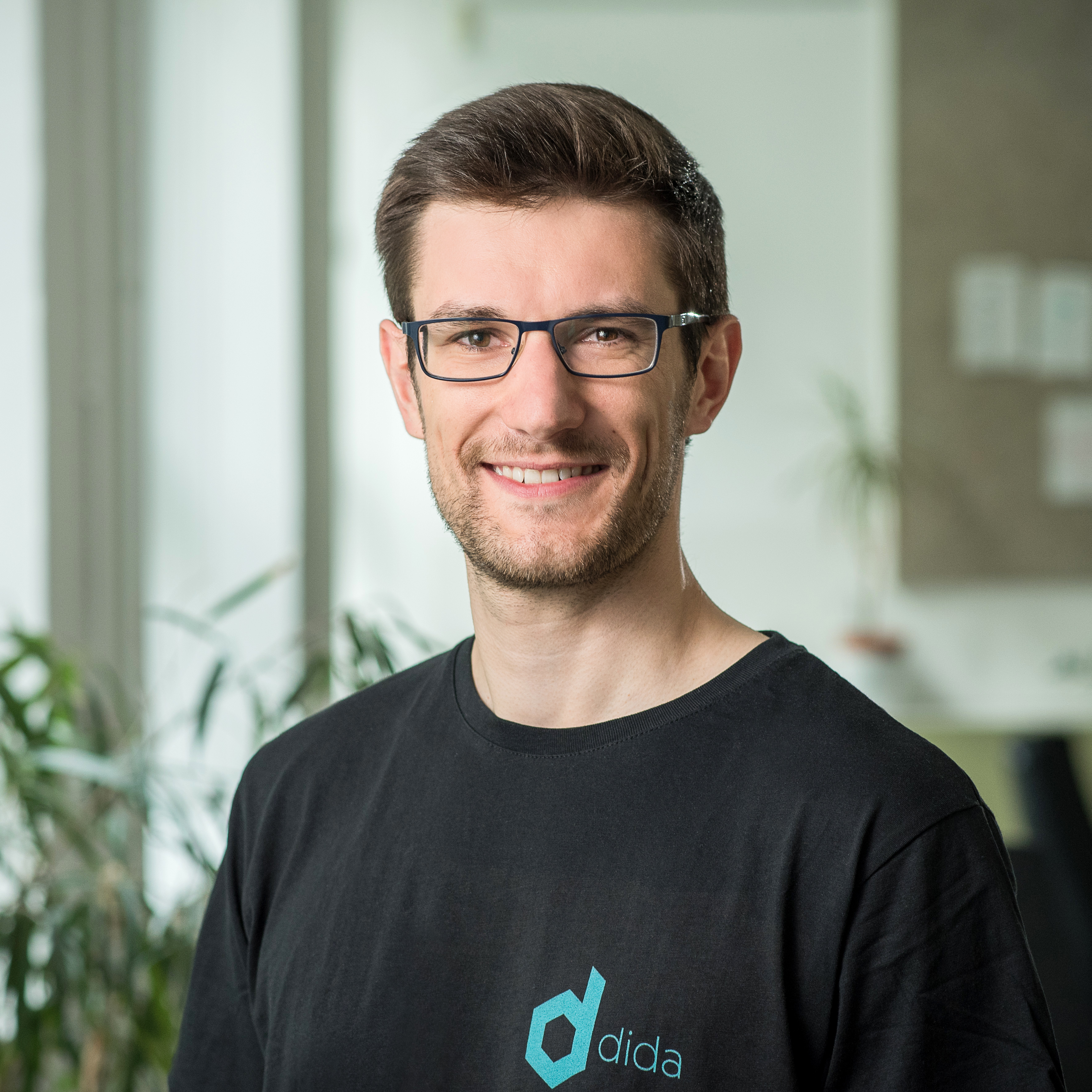
About the Co-Author
During his studies in mathematics (TU Berlin) Jan focussed on applied topics in optimization, functional analysis, and image processing. His doctoral studies (TU Berlin) explored the interplay between theoretical and empirical research on neural networks. This resulted in his PhD thesis investigating the reliability of deep learning for imaging and computer vision tasks in terms of interpretability, robustness, and accuracy. At dida he works as a Machine Learning Researcher at the interface of scientific research and software development.