Detecting Failure Modes in Image Reconstructions with Interval Neural Network Uncertainty
by Luis Oala, Cosmas Heiß, Jan Macdonald, Maximilian März, Gitta Kutyniok, Wojciech Samek
Year:
2021
Publication:
Int J CARS 16, 2089–2097
Abstract:
The quantitative detection of failure modes is important for making deep neural networks reliable and usable at scale. We consider three examples for common failure modes in image reconstruction and demonstrate the potential of uncertainty quantification as a fine-grained alarm system.
Link:
Read the paperAdditional Information
Brief introduction of the dida co-author(s) and relevance for dida's ML developments.
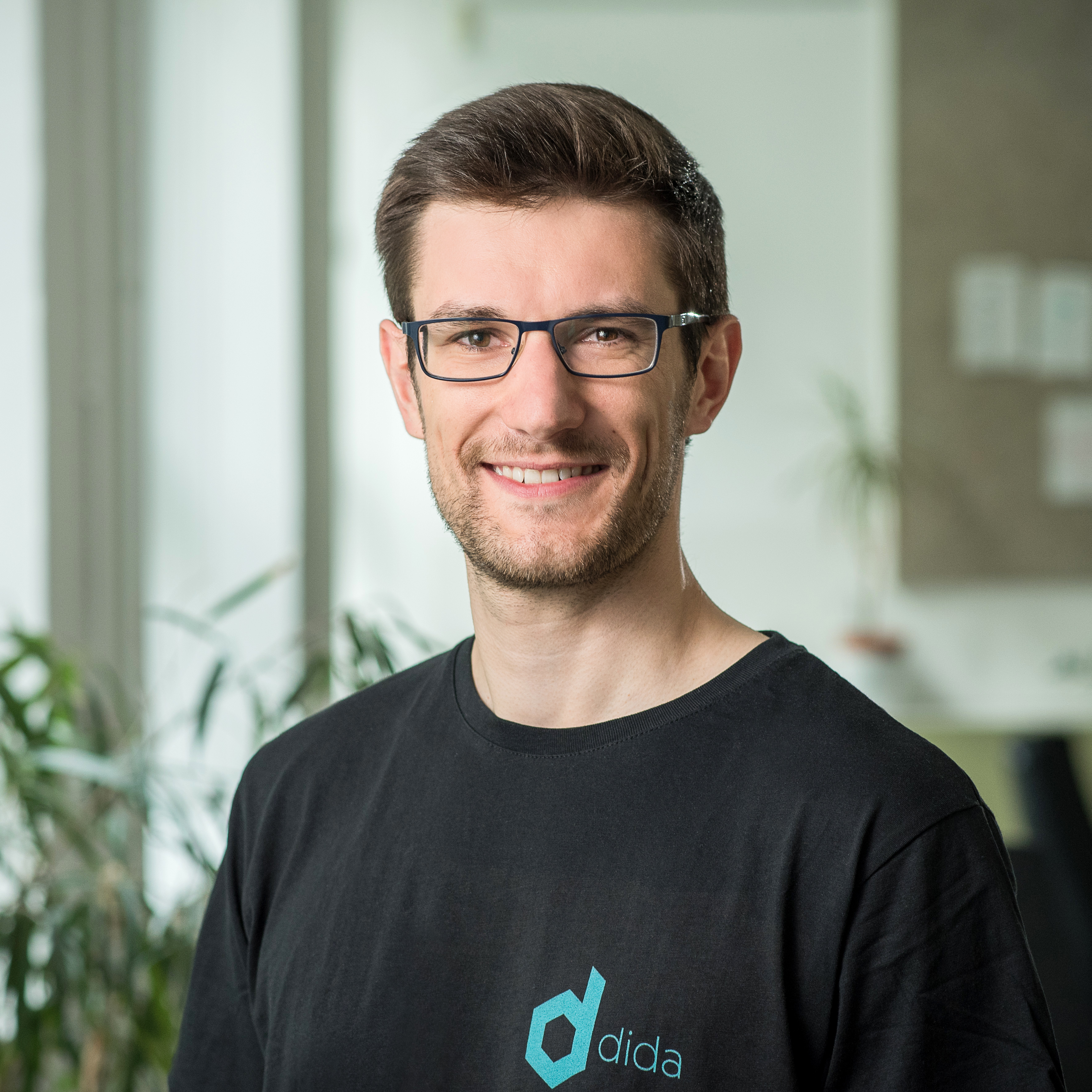
About the Co-Author
During his studies in mathematics (TU Berlin) Jan focussed on applied topics in optimization, functional analysis, and image processing. His doctoral studies (TU Berlin) explored the interplay between theoretical and empirical research on neural networks. This resulted in his PhD thesis investigating the reliability of deep learning for imaging and computer vision tasks in terms of interpretability, robustness, and accuracy. At dida he works as a Machine Learning Researcher at the interface of scientific research and software development.