Two accepted papers at ICLR
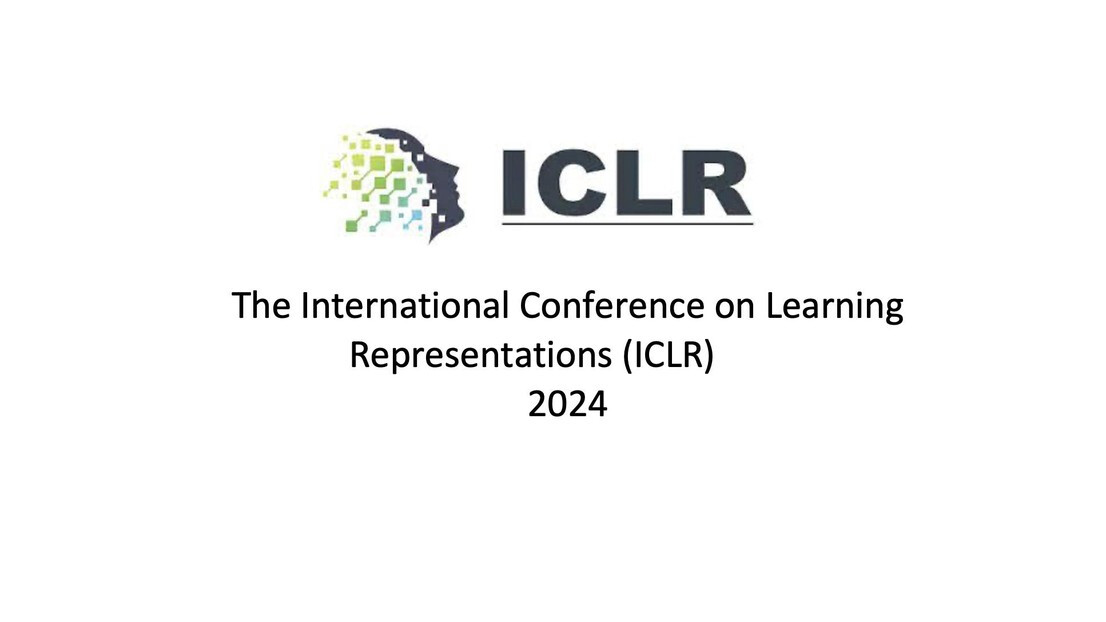
We are happy two announce that two papers with dida contribution will appear in the proceedings of this year’s International Conference on Learning Representations (ICLR), which will take place in Vienna in early May. Next to NeurIPS and ICML, the ICLR is one of the three main conference on machine learning.
The paper Improved sampling via learned diffusions investigates how sampling from specified probability densities can be approached via diffusion processes in which the drift is learned via neural networks. A perspective from measures on path space is proposed which allows to generalize existing concepts such as Schrödinger bridges or diffusion based generative modeling. This new perspective in consequence allows to design more efficient loss functions that can lead to vast improvements in numerical experiments.
The paper Fast and unified path gradient estimators for normalizing flows suggest more efficient algorithms for the variance-reduced training of normalizing flows for the sampling from complex probability distribution that for instance appear in physics or molecular dynamics. It is further derived how variance reduction methods can be applied to likelihood training which is relevant when target data samples are available.
At dida, we are very happy that we can continue our ambition to conduct cutting edge research and contribute to the machine learning community. We can therefore continue our path to combine practical machine learning with innovative research. It is the company’s philosophy that both worlds strongly benefit from one another.