Legislating AI, what makes it difficult? - A closer look at the AI Act
Liel Glaser (PhD)
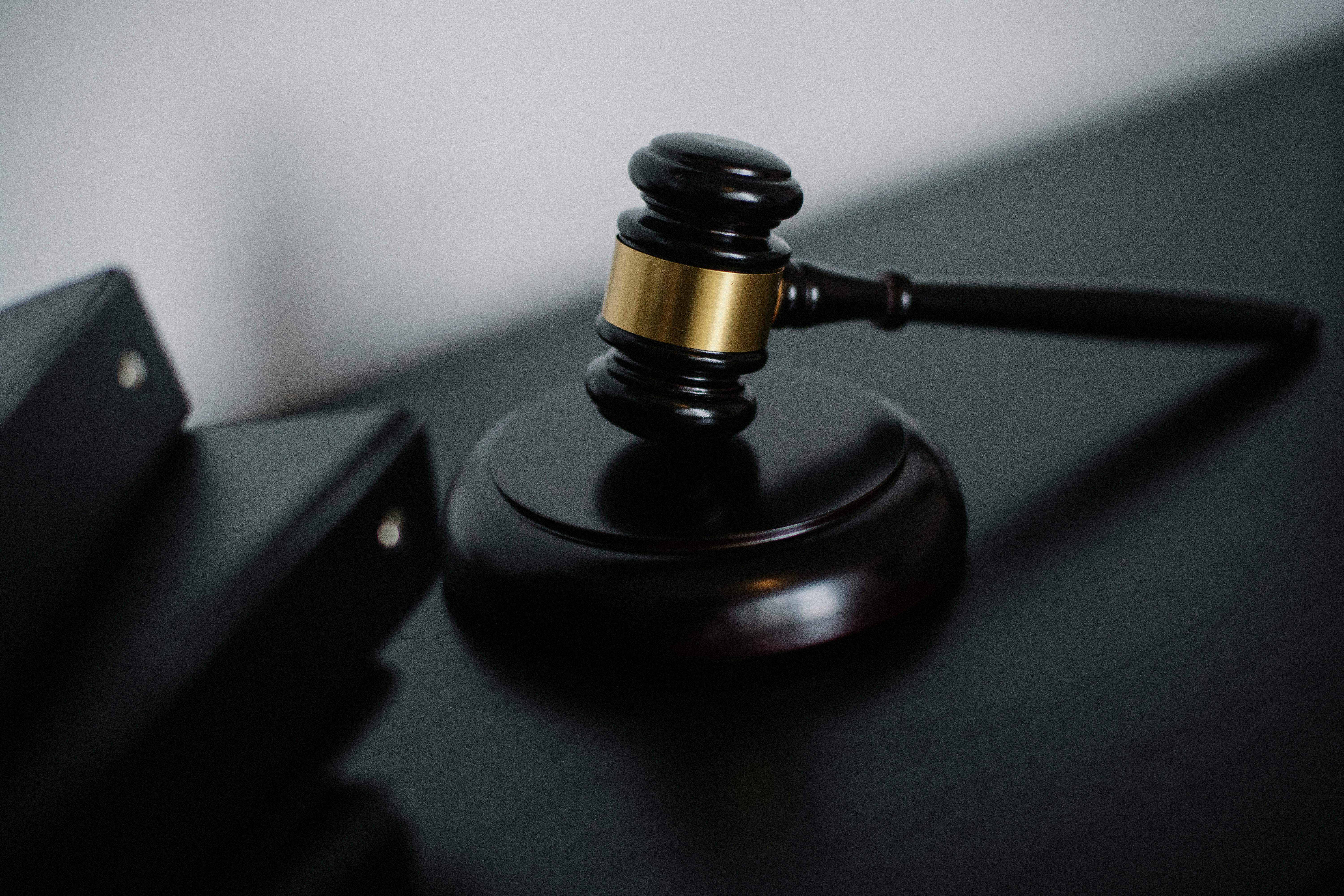
One of the biggest challenges in working on machine learning and AI is the speed at which the field is developing. New articles are published daily, and almost every week, a new model emerges that surpasses existing ones. It is difficult to predict where the next big innovation will arise and how it will be applied. The EU also faced this challenge in crafting the AI Act. How do you write a useful law that can address the misuse of technologies that do not yet exist?
To address this the EU decided to adopt a comprehensive definition of AI, focusing on a technology-agnostic description.
“AI system" means a machine-based system designed to operate with varying levels of autonomy and that may exhibit adaptiveness after deployment and that, for explicit or implicit objectives, infers, from the input it receives, how to generate outputs such as predictions, content, recommendations, or decisions that can influence physical or virtual environments.” (AI act, Article 1 paragraph 1)
In parts, this could also apply to systems already in use that are not generally considered as machine learning or AI. However, it should cover all AI systems. Wide coverage is important in this context since AI is already used in many different fields. AI is likely impacting all European citizens already, and since we cannot put the stochastic genie back in the lamp, we need to find ways to carefully consider our wishes.
Disclaimer: This article is the result of one ML Scientist taking a deep dive into EU regulations and trying to interpret and understand them. It should not be taken as legal advice and all errors are definitely my own.
The risk levels of AI
In the AI Act, AI is regulated according to the risk that a particular application represents. The four categories are unacceptable risk, high risk, transparency risk, and low risk, with separate rules applying to very large models, which they denominate as general-purpose AI models. Let us look at the models in order of risk, starting with the category of unacceptable risk.
The models that pose an unacceptable risk are generally prohibited. Broadly speaking, these are AI systems that are judged to have a harmful impact, particularly those that can impede human independence and dignity.
Categories of prohibited AI models
The first category of AI systems prohibited under the EU AI Act includes those aimed at subliminally manipulating or exploiting people's weaknesses, thereby modifying decision-making or behavior in ways that could cause significant harm. The impact of this provision may be difficult to predict, as it depends on the threshold for determining what constitutes significant harm.
Next, they address systems that classify individuals into groups based on their social behavior or personality traits -- whether known, predicted, or inferred -- and apply social scoring to them. This becomes particularly problematic if these scores lead to discrimination beyond the context in which the data was collected or if the discrimination is disproportionate to the social behavior being scored. For example, it may be permissible for a park to charge slightly more for admission if a group identified as more likely to litter is targeted, but it is not acceptable to exclude the group entirely.
Another prohibited category includes systems used to predict criminal activity or the risk of criminal activity, effectively banning predictive policing as depicted in the story "Minority Report."
The Act prohibits emotion recognition at work or school, except for safety reasons, such as monitoring when pilots become fatigued.
Additionally, it focuses on reigning in the use of biometrics, forbidding the scraping of facial recognition data from the internet or CCTV, as well as remote biometric identification in publicly accessible spaces and biometric categorization of individuals for law enforcement purposes. However, there are exceptions for the prevention of terrorism or human trafficking.
The next category is high-risk applications, which are allowed but strongly regulated. These applications either involve models used in safety components or safety-critical products or fall into one of eight other classes deemed especially risky.
High risk AI models
Safety-critical products are defined as any product already covered by EU safety laws (e.g., toys or medical devices). The eight categories considered especially risky are:
Biometrics, such as using a model for emotion detection or categorizing people by protected characteristics (e.g., age, gender, or origin)
Critical infrastructure
Education and vocational training
Employment, worker management, and access to self-employment
Access to and enjoyment of essential private services and essential public services and benefits
Law enforcement
Migration, asylum, and border control management
Administration of justice and democratic processes
This category is, however, weakened through an important exception:
Systems that fall into the second category but do not pose a significant risk of harm to the health, safety, or fundamental rights of natural persons, are not high-risk systems. (AI Act, Article 6 paragrah 3)
This exception applies if the system performs a narrow procedural task, improves the result of something a human has already done, supervises human decision-making but does not replace or influence it without human review, or if the system only performs a preparatory task for an assessment.
Overall this leads to a very high threshold for a system to be considered high risk. The closest to a high-risk system any past dida use case comes is our project on Smart Access Control with Facial Recognition.
This system does use biometric data, however, the task performed is very narrow, namely checking the database of registered users against the facial images, and does not pose a risk of harm to fundamental rights, hence the system itself is of low risk.
Systems that fall into the high-risk category must undergo a conformity assessment procedure before they can be sold or used in the EU. Providers will need to meet a range of requirements, starting with data quality (e.g., no copyright-infringing materials, fair representation of relevant groups in the data, etc.), and extending to the system’s robustness and cybersecurity measures. In some cases, providers will also need to conduct a fundamental rights impact assessment to demonstrate that the system complies with EU law.
The exact details of these requirements will be determined by an advisory board, which will also develop harmonized standards. Compliance with these standards will grant providers a presumption of conformity. Once a system is on the market, providers must continue monitoring it and take corrective actions if necessary.
This risk monitoring system must cover the entire lifecycle of the high-risk AI system and track known and reasonably foreseeable risks that the system may pose to health, safety, or fundamental rights.
Transparency and low risk models
The transparency risk category primarily applies to chatbots, such as those commonly used in customer service today. Although the risk to individuals interacting with these chatbots is generally low, it remains important that people are aware when they are engaging with a model rather than a human. To ensure this, the AI Act mandates that users must be informed when interacting with a chatbot, and that all AI-generated and manipulated content must be clearly marked as such. Providers are responsible for this marking and must also provide methods of detection.
One could argue that this law indicates that LLMs (Large Language Models) have passed the Turing Test; otherwise, it would not be necessary for chatbots to disclose that they are not human, as users would be able to tell. At dida, we develop chatbots and Retrieval-Augmented Generation (RAG) systems (find more information about RAG systems at our LLM overview or this blog article), ensuring that all data sources are transparently disclosed to users.
Everything not covered in one of the categories above will be considered low risk and not directly affected by the regulation. Effectively, most existing applications of AI should fall into this category. However, many of the requirements, such as good data stewardship, robust code, and cybersecurity, are hallmarks of well-developed models. Hence, AI developers are encouraged to voluntarily comply with the harmonized standards even when developing low risk systems.
Large models a class of their own
Outside of all risk categories the EU defines general-purpose AI models,
[...] an AI model, including where such an AI model is trained with a large amount of data using self-supervision at scale, that displays significant generality and is capable of competently performing a wide range of distinct tasks [..] (AI Act, Article 3 paragraph 63)
The EU Commission assumes that these very large models have the potential to cause systemic risks. They set the threshold that a model trained with more than 1025 FLOPS of computation time is presumed to hold systemic risk, and therefore providers must notify the EU Commission about the model. All general-purpose models are required to meet documentation standards that ensure certain information is available to the AI office upon request, as well as to providers planning to integrate the system into their AI frameworks.
They must also comply with all applicable EU copyright laws. However, these regulations are waived if the model does not pose a systemic risk and is released under an open-source license that permits reuse and modifications. Providers of models deemed to hold systemic risk are additionally obligated to continuously assess and mitigate the risks their model presents.
How will the Act come to life?
Overall, the EU AI Act is an attempt to regulate a field that has been growing quickly and that can immensely benefit or harm people, often at the same time. The AI Act aims to strike a difficult balance between being rigorous enough to protect individuals while not being so rigid as to stifle development. How well this balance is achieved will depend on the many examples that are being worked out and on how the courts decide to apply the law. The AI Act also promises more concrete examples in the future, with expert groups, such as the EU AI Pact, being assembled to provide these details.
Another important aspect of the law is the question of who is responsible for a given AI model. The EU Act splits this responsibility between providers and deployers. Providers are entities that develop AI, or have AI developed for them, and then put the system into service under their own name. Deployers, on the other hand, are entities using an AI system. This division is somewhat subtle since a company deploying a custom solution (e.g., one developed by dida) would be considered a provider while buying an off-the-shelf solution that performs similar functions would merely make them a deployer.
A good model is reliable, transparent, and understandable
At dida, we subscribe to many of the expectations set forth by the AI Act as a matter of course. We understand that transparency regarding why a model makes certain predictions is important to our customers. While there are limitations to this, we are always excited to push the boundaries. We primarily work with open-source models or models we train ourselves, ensuring that the customer has full control.
Whenever possible, the data we use comes directly from the customer, which not only tailors the model perfectly to your use case but also ensures we are aware of copyright information and other potential issues that can arise from data scraped from the internet. We invite you to check out our blog post on "Data Privacy: Machine Learning and the GDPR" to learn more about how we handle data privacy and compliance in our practices.
Read more about AI, Machine Learning & related aspects:
AI industry projects: Find out which projects dida has implemented in the past and how these AI solutions have helped companies to achieve more efficient processes.
AI knowledge base: Learn more about various aspects of AI, AI projects and process automation.
dida team: Get to know the people and company behind an AI company - their background and profiles.
Contact
If you would like to speak with us about this topic, please reach out and we will schedule an introductory meeting right away.